Migration learning method for heterogeneous users
A transfer learning and user-oriented technology, which is applied in the field of transfer learning for heterogeneous users, can solve the problems of effect impact, accuracy cannot be guaranteed, and classification accuracy is difficult to guarantee, so as to reduce the risk of privacy leakage and meet the requirements of high classification accuracy , the effect of improving the classification accuracy
- Summary
- Abstract
- Description
- Claims
- Application Information
AI Technical Summary
Problems solved by technology
Method used
Image
Examples
Embodiment Construction
[0043] In order to make the purpose, technical solutions and advantages of the embodiments of the present application clearer, the technical solutions in the embodiments of the present application will be clearly and completely described below in conjunction with the drawings in the embodiments of the present application.
[0044] Such as figure 1 or figure 2 As shown, the present invention discloses a transfer learning method for heterogeneous users, which includes the following steps:
[0045] Step 1. Participants perform data collection and primary processing on the local side to achieve the first data dimensionality reduction.
[0046] Specifically, step 1 includes the following steps:
[0047] Step 1. Participants perform data collection and primary processing on the local side to achieve the first data dimensionality reduction.
[0048] Step 2. According to the requirements of the participants, the server side selects and demarcates the source domain and the target d...
PUM
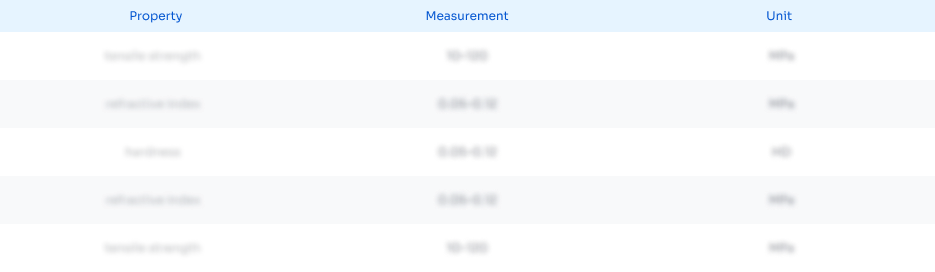
Abstract
Description
Claims
Application Information
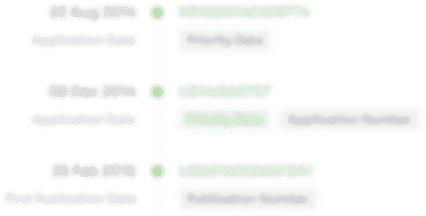
- R&D
- Intellectual Property
- Life Sciences
- Materials
- Tech Scout
- Unparalleled Data Quality
- Higher Quality Content
- 60% Fewer Hallucinations
Browse by: Latest US Patents, China's latest patents, Technical Efficacy Thesaurus, Application Domain, Technology Topic, Popular Technical Reports.
© 2025 PatSnap. All rights reserved.Legal|Privacy policy|Modern Slavery Act Transparency Statement|Sitemap|About US| Contact US: help@patsnap.com