Image Classification Model and Its Classification Method Based on Hybrid Depth Separable Dilated Convolution
A classification model and deep technology, applied in the field of image processing, can solve the problems of losing the detailed information of features, reducing the classification accuracy, difficult to optimize, etc., to achieve the effect of supplementing the context information, improving the classification accuracy, and improving the expression ability.
- Summary
- Abstract
- Description
- Claims
- Application Information
AI Technical Summary
Problems solved by technology
Method used
Image
Examples
Embodiment
[0040] Such as Figure 1 to Figure 2 As shown, this embodiment provides an image classification method based on mixed depth separable dilated convolution, which adds a mixed depth separable dilated convolution module to the built network model structure, which consists of multiple depths with different dilation rates The composition of the dilated convolutional layer can be separated to expand the scope of feature extraction, supplement the context information of the feature, improve the expressive ability of the network model, and thus improve the classification accuracy of the model.
[0041] Specifically, the image classification model of this embodiment includes a convolutional layer packed from front to back, a batch normalization layer, a rectified linear unit layer, 2 hybrid depthwise separable dilated convolution modules, and a maximum pooling layer , 3 mixed depth separable dilation convolution modules, 1 max pooling layer, 3 mixed depth separable dilation convolution...
PUM
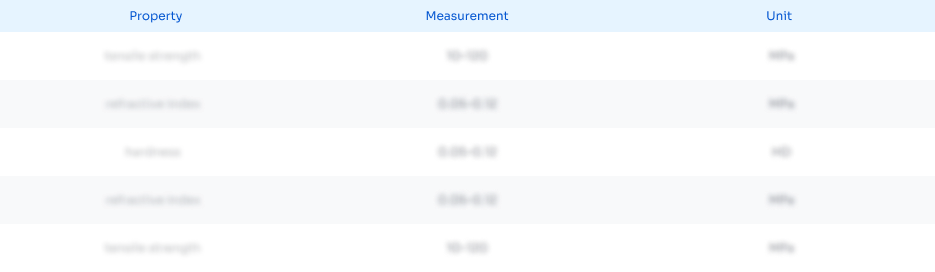
Abstract
Description
Claims
Application Information
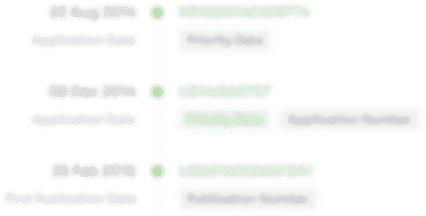
- R&D Engineer
- R&D Manager
- IP Professional
- Industry Leading Data Capabilities
- Powerful AI technology
- Patent DNA Extraction
Browse by: Latest US Patents, China's latest patents, Technical Efficacy Thesaurus, Application Domain, Technology Topic, Popular Technical Reports.
© 2024 PatSnap. All rights reserved.Legal|Privacy policy|Modern Slavery Act Transparency Statement|Sitemap|About US| Contact US: help@patsnap.com