Two-stage expression animation generation method based on dual generative adversarial network
A stage and network technology, applied in the field of two-stage expression animation generation, can solve problems such as unreasonable artifacts, low generation results, and blurred resolution of generated images
- Summary
- Abstract
- Description
- Claims
- Application Information
AI Technical Summary
Problems solved by technology
Method used
Image
Examples
Embodiment 1
[0087] The two-stage expression animation generation method based on the dual generation confrontation network of the present embodiment, the specific steps are as follows:
[0088] The first step is to obtain the facial expression contour map of each frame of image in the dataset:
[0089] Collect the facial expression video sequence data set, use the Dlib machine learning library to extract the face in each frame of the video sequence, and simultaneously obtain 68 feature points in each face (in the field of expression transfer, 68 feature points constitute Contours of faces, eyes, mouths, and noses, and 5 or 81 feature points can also be set.), such as figure 2 As shown in the odd-numbered lines, and then use line segments to connect the feature points in sequence to obtain the expression contour map of each frame of the video sequence, as shown in figure 2 Shown in the even-numbered row, recorded as e=(e 1 ,e 2 ,···,e i ,···,e n ), where e represents the collection ...
PUM
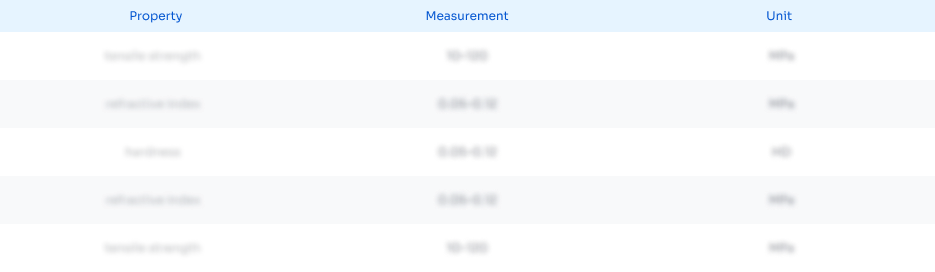
Abstract
Description
Claims
Application Information
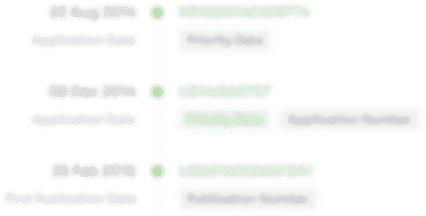
- R&D Engineer
- R&D Manager
- IP Professional
- Industry Leading Data Capabilities
- Powerful AI technology
- Patent DNA Extraction
Browse by: Latest US Patents, China's latest patents, Technical Efficacy Thesaurus, Application Domain, Technology Topic, Popular Technical Reports.
© 2024 PatSnap. All rights reserved.Legal|Privacy policy|Modern Slavery Act Transparency Statement|Sitemap|About US| Contact US: help@patsnap.com