Complex working condition bearing fault diagnosis method based on meta-learning under small sample
A technology for complex working conditions and fault diagnosis, which is used in neural learning methods, testing of mechanical components, and pattern recognition in signals to achieve fast and accurate bearing fault diagnosis, reduce costs, and reduce dependencies.
- Summary
- Abstract
- Description
- Claims
- Application Information
AI Technical Summary
Problems solved by technology
Method used
Image
Examples
Embodiment 1
[0038] Embodiment 1: The present invention is based on a shallow convolutional neural network, and utilizes a meta-learning strategy in the meta-training process to obtain general knowledge of bearing faults by learning and optimizing models for multiple tasks, which can be achieved by using the acquired knowledge meta-test stage Fast and accurate bearing fault diagnosis under small samples under new working conditions, the bearing fault diagnosis method specifically includes the following steps:
[0039] Step 1: Use the acceleration sensor to collect and obtain the vibration signal data of the bearing under different working conditions, and perform normalization processing;
[0040] Step 2: Use the short-time Fourier transform to convert the original vibration signal of the bearing into a time-frequency image to obtain the comprehensive fault information of the bearing in the time domain and frequency domain, and construct a meta-learning data set;
[0041] Step 3: Divide the...
Embodiment 2
[0047] Example 2: The used bearing fault data set has three operating conditions (different loads and rotating speeds), each operating condition includes normal, inner ring fault, outer ring fault and ball fault, and each fault has three There are a total of 10 bearing operating states for different fault sizes, each operating state includes 120 time-frequency diagrams, and a total of 1200 time-frequency diagrams under each working condition. Based on the meta-learning training strategy, a 10-way 1( / 5)shot fault classification task is constructed. A total of 200 tasks are randomly sampled during meta-training and 100 tasks are randomly sampled during meta-testing. By performing meta-training on one working condition, meta-testing on other different working conditions.
[0048] refer to figure 1 , the bearing fault diagnosis method of the present invention comprises the following steps:
[0049] Step 1: Use the acceleration sensor to collect and obtain the vibration signal d...
PUM
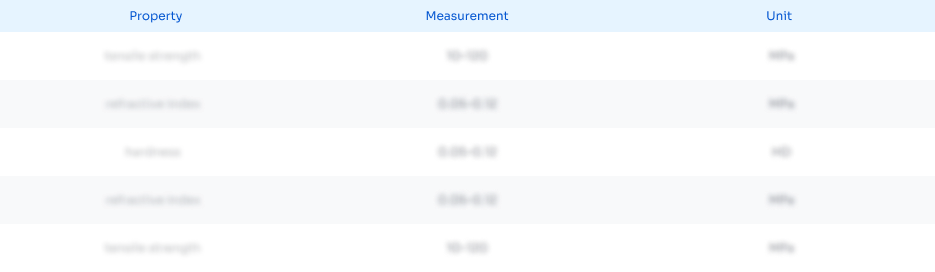
Abstract
Description
Claims
Application Information
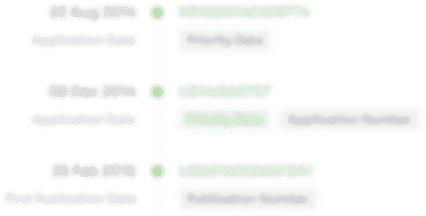
- R&D Engineer
- R&D Manager
- IP Professional
- Industry Leading Data Capabilities
- Powerful AI technology
- Patent DNA Extraction
Browse by: Latest US Patents, China's latest patents, Technical Efficacy Thesaurus, Application Domain, Technology Topic, Popular Technical Reports.
© 2024 PatSnap. All rights reserved.Legal|Privacy policy|Modern Slavery Act Transparency Statement|Sitemap|About US| Contact US: help@patsnap.com