Bearing fault classification method based on CNN and Adaboost
A bearing and weak classification technology, applied in the field of UAV bearing fault classification based on CNN and Adaboost integrated learning, can solve the problem that the model accuracy is easily affected by the cardinality, does not consider the signal fault correlation, model training efficiency and fault diagnosis accuracy Low-level problems, to achieve the effect of improving recognition accuracy and prosperous ability, conducive to real-time performance, and flexible structure
- Summary
- Abstract
- Description
- Claims
- Application Information
AI Technical Summary
Problems solved by technology
Method used
Image
Examples
Embodiment
[0066] For the convenience of description, the relevant technical terms appearing in the specific implementation are explained first:
[0067] EMD (Empirical Mode Decomposition): Empirical Mode Decomposition;
[0068] VMD (variational mode decomposition) variational mode decomposition;
[0069] Adaboost (Adaptive Boosting): A type of integrated learning boosting;
[0070] CNN: convolutional neural network;
[0071] ANN (Artificial Neutral Network): artificial neural network;
[0072] RNN (Recurrent Neural Networks): recurrent neural network;
[0073] figure 1 It is a flow chart of the present invention's bearing fault classification method based on CNN and Adaboost.
[0074] In this example, if figure 1 Shown, a kind of bearing fault classification method based on CNN and Adaboost of the present invention comprises the following steps:
[0075] S1. Obtain signal data set
[0076] Obtain all bearing signals in the UAV to form a signal data set F={f (i) |i∈[1,m]}, f (i...
example
[0131] Suppose a UAV has n bearings, which are f1, f2,..., fn. For f1, firstly, the f1 bearing signal is decomposed into time-domain signal S1 and time-frequency signal F1 based on variational mode decomposition (VMD), and then the fault diagnosis of f1 is carried out through the integrated model CNN+Adaboost. Then based on the above process, make fault diagnosis for bearings f2...fn respectively, the diagnosis process is as follows Figure 7 As shown in Fig. 1, the fault condition of the bearing is finally judged according to the diagnosis result of each bearing.
[0132] Model evaluation parameters accuracy Acc, precision rate P, recall rate R, F1 and calculation speed and other indicators.
[0133]
[0134]
[0135]
[0136]
[0137] Let S N and F N Represent the number of time-domain models and time-frequency domain models respectively, and the final test results are shown in Table 1.
[0138] index parameter S N
F N
ACC(%) P(%) R(%) ...
PUM
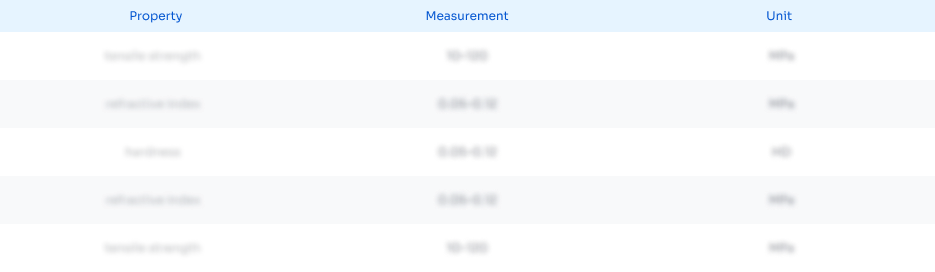
Abstract
Description
Claims
Application Information
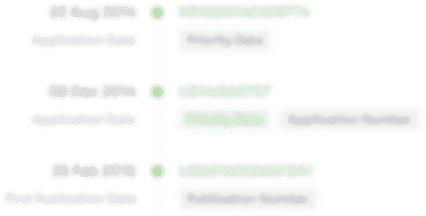
- R&D Engineer
- R&D Manager
- IP Professional
- Industry Leading Data Capabilities
- Powerful AI technology
- Patent DNA Extraction
Browse by: Latest US Patents, China's latest patents, Technical Efficacy Thesaurus, Application Domain, Technology Topic, Popular Technical Reports.
© 2024 PatSnap. All rights reserved.Legal|Privacy policy|Modern Slavery Act Transparency Statement|Sitemap|About US| Contact US: help@patsnap.com