Method for quickly mixing high-order attention domain adversarial network based on transfer learning
A technology of transfer learning and attention, applied in the field of neural network deep learning, can solve problems such as insufficient capture of complex high-order saliency information, improve generalization ability and classification accuracy, avoid negative transfer, and improve transferability sexual effect
- Summary
- Abstract
- Description
- Claims
- Application Information
AI Technical Summary
Problems solved by technology
Method used
Image
Examples
Embodiment 1
[0041] Embodiment 1: The content of the invention is further explained in conjunction with the accompanying drawings and the unsupervised domain adaptation on the data set Digits, a method for a fast hybrid high-order attention domain adversarial network based on transfer learning, figure 1 is a block diagram of a fast hybrid high-order attention and domain confrontation adaptive model according to an embodiment of the present invention;
[0042] The method includes the following steps:
[0043] Step1: Divide the image dataset to be processed into source domain and target domain;
[0044] Step2: Design a fast hybrid high-order attention and domain adversarial adaptive network FHAN for the image dataset to be processed. The fast hybrid high-order attention includes channel attention and high-order spatial attention, and the domain adversarial adaptive network includes features Extractor G, Domain Discriminator D, Classifier C;
[0045] Step3: Preprocess the source domain and ...
PUM
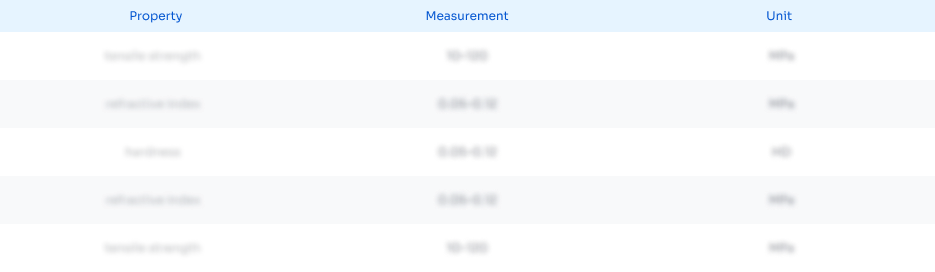
Abstract
Description
Claims
Application Information
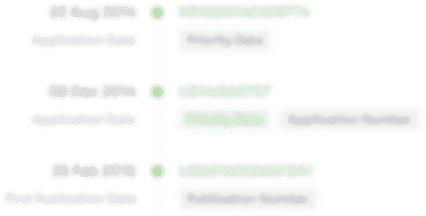
- R&D
- Intellectual Property
- Life Sciences
- Materials
- Tech Scout
- Unparalleled Data Quality
- Higher Quality Content
- 60% Fewer Hallucinations
Browse by: Latest US Patents, China's latest patents, Technical Efficacy Thesaurus, Application Domain, Technology Topic, Popular Technical Reports.
© 2025 PatSnap. All rights reserved.Legal|Privacy policy|Modern Slavery Act Transparency Statement|Sitemap|About US| Contact US: help@patsnap.com