Surface defect detection method based on multi-scale convolution and trilinear global attention
A defect detection and attention technology, applied in neural learning methods, character and pattern recognition, details involving image stitching, etc., can solve problems such as inability to meet needs, large differences in defect size, industrial environment background, etc. Strong response, enhanced effect of difference
- Summary
- Abstract
- Description
- Claims
- Application Information
AI Technical Summary
Problems solved by technology
Method used
Image
Examples
Embodiment 1
[0035] The embodiment of the present invention describes a surface defect detection method based on multi-scale convolution and trilinear global attention, such as figure 1 shown.
[0036] The whole network consists of four parts: feature fusion network module, codec network module, trilinear global attention module and finally decision module. Among them, the first feature fusion network module fuses shallow and deep features to provide multi-level semantic information for the codec module, which has a strong ability to capture local fine-grained features; the second codec network module multiplexes convolution After the features of the corresponding scale realize the recovery of semantic information; the third trilinear global attention module obtains the importance of each feature channel through the adaptive learning method, learns the correlation between features, and improves useful features according to the importance And suppress features that are not very useful for ...
Embodiment 2
[0058] The scheme in embodiment 1 is verified below in conjunction with concrete experiment, see description below for details:
[0059] 1. Experimental settings
[0060] Datasets and evaluation metrics:
[0061] (1) Kolektor SDD dataset
[0062] The KolektorSDD surface defect dataset used for this training and evaluation is the surface crack image of the electronic steering gear provided and annotated by the Kolektor Group (http: / / www.vicos.si / Downloads / KolektorSDD), and the dataset was collected in an industrial environment , with a resolution of 1408*512 pixels, such as Figure 5 shown. More specifically, the dataset consists of 50 samples of defective electronic diverters, each with up to 8 surfaces. This results in a total of 399 images, of which 52 are clearly visible defects and are presented as Positive samples, the remaining 347 pictures are non-defective negative samples, the number of positive samples in the training set is 26, and the number of negative samples...
PUM
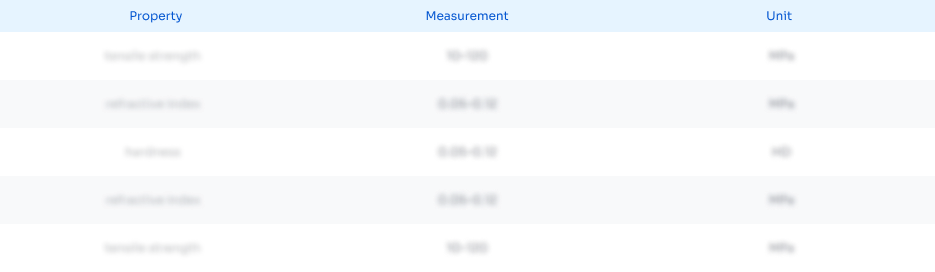
Abstract
Description
Claims
Application Information
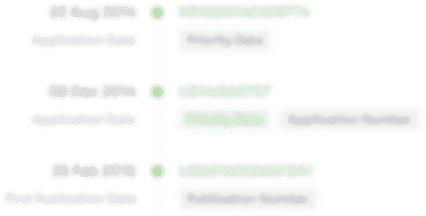
- R&D Engineer
- R&D Manager
- IP Professional
- Industry Leading Data Capabilities
- Powerful AI technology
- Patent DNA Extraction
Browse by: Latest US Patents, China's latest patents, Technical Efficacy Thesaurus, Application Domain, Technology Topic.
© 2024 PatSnap. All rights reserved.Legal|Privacy policy|Modern Slavery Act Transparency Statement|Sitemap