Video question-answering method based on progressive graph attention network
An attention and network technology, applied in the field of video question answering, can solve problems such as low accuracy
- Summary
- Abstract
- Description
- Claims
- Application Information
AI Technical Summary
Problems solved by technology
Method used
Image
Examples
example
[0164] After experiments, it was found that the two multi-choice (Multi-Choice) sub-datasets in the existing large-scale video question answering dataset TGIF-QA have serious answer bias. These biases can have a great impact on the accuracy of the model. In order to solve this problem, this example builds a new dataset TGIF-QA-R on the basis of TGIF-QA. In this data set, candidate answers are independent of each other, and in this way, the impact caused by answer bias can be effectively reduced.
[0165] Test the effect of this method on three large benchmark data sets TGIF-QA, MSVD-QA and MSRVTT-QA and the newly constructed TGIF-QA-R data set, as can be seen from the experimental effect, the method proposed by the present invention is superior method at the highest level.
[0166] 1. Test results on TGIF-QA and TGIF-QA-R datasets
[0167]
[0168]
[0169] Table 1
[0170] It can be concluded from Table 1 that the present invention has achieved the best performance ...
PUM
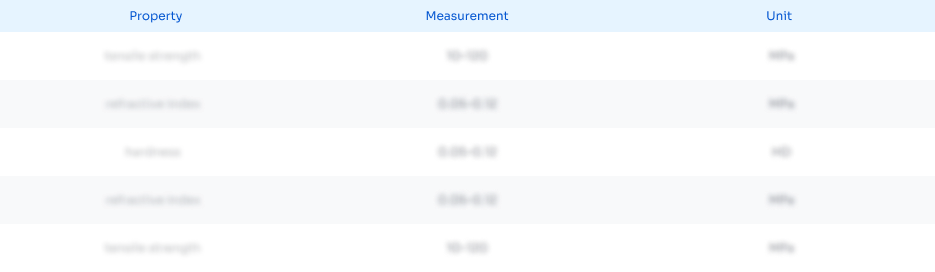
Abstract
Description
Claims
Application Information
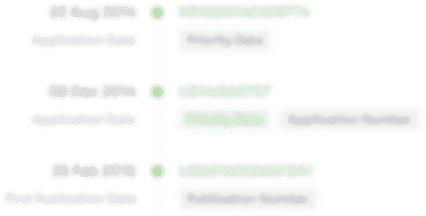
- R&D
- Intellectual Property
- Life Sciences
- Materials
- Tech Scout
- Unparalleled Data Quality
- Higher Quality Content
- 60% Fewer Hallucinations
Browse by: Latest US Patents, China's latest patents, Technical Efficacy Thesaurus, Application Domain, Technology Topic, Popular Technical Reports.
© 2025 PatSnap. All rights reserved.Legal|Privacy policy|Modern Slavery Act Transparency Statement|Sitemap|About US| Contact US: help@patsnap.com