Integrated unsupervised student behavior clustering method
A clustering method and unsupervised technology, applied in the direction of instruments, character and pattern recognition, data processing applications, etc., can solve problems such as inability to meet real-time applications, inability to obtain student label information, and error in analysis results
- Summary
- Abstract
- Description
- Claims
- Application Information
AI Technical Summary
Problems solved by technology
Method used
Image
Examples
Embodiment Construction
[0024] 1. Collect student behavior data. The student behavior data is described as follows:
[0025] (1) Consumer behavior data. The data includes three attributes: time, location, and consumption amount. According to the consumption time and consumption location, the consumption behavior data is further divided into breakfast behavior data, lunch behavior data, dinner behavior data and shopping behavior data. Among them, the consumption time periods of the three meals are respectively stipulated as 6:00am to 9:00am, 11:00am to 2:00pm, and 4:30pm to 8:30pm. The time period of the shopping behavior is defined as the whole day.
[0026] (2) Behavioral data entering the library. Due to the small number of libraries, the location of the behavior is no longer included, and only the entry time is included.
[0027] (3) Log in the behavior data of the gateway system. The gateway system is a protocol converter deployed between the Internet and the campus LAN. When students access ...
PUM
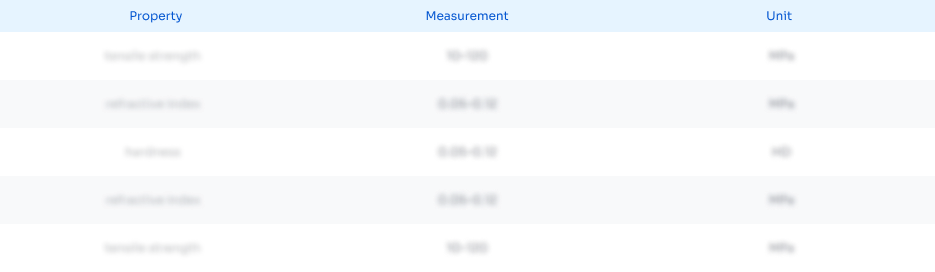
Abstract
Description
Claims
Application Information
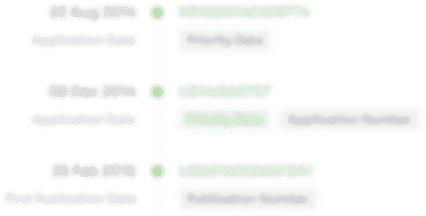
- R&D
- Intellectual Property
- Life Sciences
- Materials
- Tech Scout
- Unparalleled Data Quality
- Higher Quality Content
- 60% Fewer Hallucinations
Browse by: Latest US Patents, China's latest patents, Technical Efficacy Thesaurus, Application Domain, Technology Topic, Popular Technical Reports.
© 2025 PatSnap. All rights reserved.Legal|Privacy policy|Modern Slavery Act Transparency Statement|Sitemap|About US| Contact US: help@patsnap.com