Cross-model face feature vector conversion system and method
A face feature and conversion system technology, applied in the field of face recognition, can solve the problems of uncommon face feature vector, ungeneric feature vector, waste of human resources, etc., so as to improve performance and generalization, and improve feature expression ability. , the effect of improving the conversion success rate
- Summary
- Abstract
- Description
- Claims
- Application Information
AI Technical Summary
Problems solved by technology
Method used
Image
Examples
Embodiment 1
[0034] A conversion system for cross-model face feature vectors, including a data extraction module, a network training module, and a conversion module.
[0035] The data extraction module is used to collect face image data sets, source feature vectors and target feature vectors; mark the identity category of the face image data sets and form a training data set, and use the face image data and source feature vectors as training samples, And the labeled identity category is used as the real label value;
[0036] The network training module is used to input the input samples in the training data set in the data extraction module into the network model for training to obtain the trained network model;
[0037] The conversion module is used for inputting the human face feature vector to be converted into the network model trained in the network training module, and outputting the converted human face feature vector.
[0038] Such as figure 1As shown, the network model is compos...
Embodiment 2
[0041] This embodiment is optimized on the basis of Embodiment 1. The network part of the deformed attention mechanism first uses the convolution layer to down-sample the image to improve semantics; then uses the multi-head attention mechanism module to slice the convolution features to The relationship information between each feature is extracted; finally, the batch normalization layer, the fully connected layer, and the activation function layer are sequentially processed and then partially spliced and fused with the convolutional network.
[0042] The present invention proposes to introduce the deformed attention mechanism into the conversion process of the face feature vector. By building the network part of the deformed attention mechanism, the local information and global information available in the face image can be fully extracted, and the feature expression ability of the model is greatly improved. , to improve the performance and generalization of the model.
[0...
Embodiment 3
[0045] This embodiment is optimized on the basis of embodiment 2, such as figure 2 As shown, the network part of the deformed attention mechanism consists of the first integration module, the position embedding vector layer, the second integration module, the batch normalization layer, the fully connected layer, the activation function layer, and the fully connected layer arranged in sequence from front to back , the activation function layer is encapsulated and obtained; the first integrated module and the second integrated module are respectively provided with several; the first integrated module and the position embedding vector layer are added and connected to the second integrated module; the first integrated module The module consists of a convolutional layer, a batch normalization layer, and an activation function layer arranged in sequence from front to back. The second integrated module consists of a reorganization vector layer, a multi-head attention mechanism module...
PUM
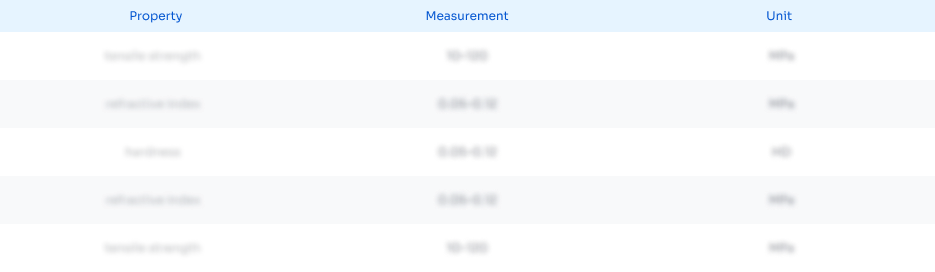
Abstract
Description
Claims
Application Information
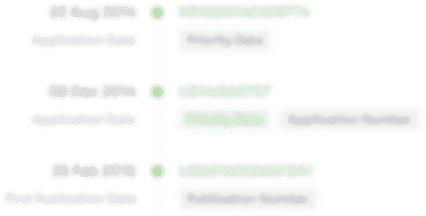
- R&D Engineer
- R&D Manager
- IP Professional
- Industry Leading Data Capabilities
- Powerful AI technology
- Patent DNA Extraction
Browse by: Latest US Patents, China's latest patents, Technical Efficacy Thesaurus, Application Domain, Technology Topic, Popular Technical Reports.
© 2024 PatSnap. All rights reserved.Legal|Privacy policy|Modern Slavery Act Transparency Statement|Sitemap|About US| Contact US: help@patsnap.com