Electrocardiosignal graph classification method based on deep learning
A technology of electrocardiogram and deep learning, applied in neural learning methods, medical science, instruments, etc., can solve problems such as poor classification and poor generalization ability of automatic classification algorithms, and achieve high classification performance
- Summary
- Abstract
- Description
- Claims
- Application Information
AI Technical Summary
Problems solved by technology
Method used
Image
Examples
specific Embodiment approach 1
[0018] A kind of classification method based on the electrocardiogram of deep learning of the present embodiment, such as Figure 5 As shown, the method is realized through the following steps:
[0019] Step 1, preprocessing the image data of the collected electrocardiogram; wherein the preprocessing includes the steps of wavelet denoising of the image data of the electrocardiogram and data enhancement of the image data of the electrocardiogram;
[0020] Step 2. Design a convolutional neural network;
[0021] Step 3, using the designed convolutional neural network to classify the electrocardiogram.
specific Embodiment approach 2
[0023] The difference from the first embodiment is that in this embodiment, a deep learning-based classification method for electrocardiograms, in the first step, the wavelet denoising process of the image data of the electrocardiograms is specifically:
[0024] The wavelet method is used for noise reduction, and the wavelet coefficients are analyzed, the coefficients with smaller absolute values are set to 0, and the coefficients with larger absolute values are retained or shrunk, and then the processed wavelet coefficients are reconstructed to obtain the denoising signal. Depend on figure 1 It can be seen that the wavelet transform method eliminates the noise relatively well while retaining the useful signal.
specific Embodiment approach 3
[0026] The difference from the second specific embodiment is that in this embodiment, a deep learning-based classification method of ECG diagrams, in the first step, the step of data enhancement of the image data of ECG diagrams is specifically as follows:
[0027] Because the amount of sample data contained in the MIT-BIH arrhythmia data set itself used in the present invention is not large enough, in order to ensure that all sample data can be fully utilized, the data equalization method is not to under-sample a large amount of data samples, but to The oversampling operation is used for small data volume samples. First, the cardiac beat frequency of small data volume samples is expanded to any frequency between 60-120bpm, and then the original ECG data is sampled according to the randomly expanded frequency using the second-order interpolation algorithm. , the specific calculation process is as follows:
[0028]
[0029]
[0030]
[0031] Among them, L i The length...
PUM
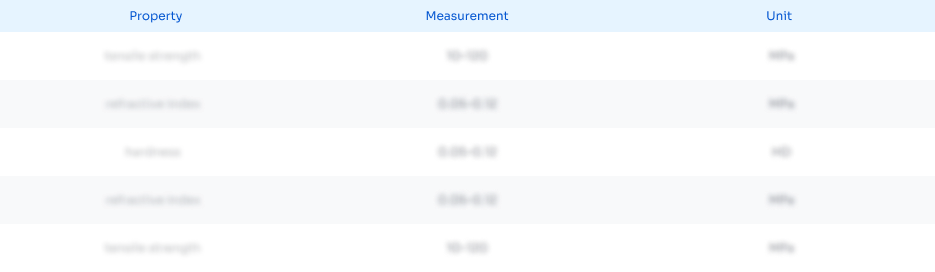
Abstract
Description
Claims
Application Information
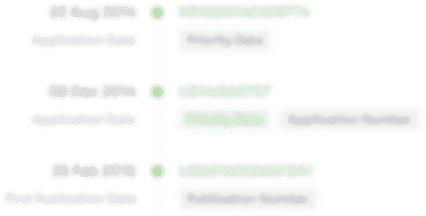
- R&D
- Intellectual Property
- Life Sciences
- Materials
- Tech Scout
- Unparalleled Data Quality
- Higher Quality Content
- 60% Fewer Hallucinations
Browse by: Latest US Patents, China's latest patents, Technical Efficacy Thesaurus, Application Domain, Technology Topic, Popular Technical Reports.
© 2025 PatSnap. All rights reserved.Legal|Privacy policy|Modern Slavery Act Transparency Statement|Sitemap|About US| Contact US: help@patsnap.com