Hyperspectral target tracking method based on feature extraction and weight coefficient parameter updating
A weight coefficient and target tracking technology, applied in the field of image processing, can solve problems such as estimation error, large amount of calculation, and poor real-time performance
- Summary
- Abstract
- Description
- Claims
- Application Information
AI Technical Summary
Problems solved by technology
Method used
Image
Examples
Embodiment Construction
[0074] The present invention is described in further detail now in conjunction with accompanying drawing.
[0075] Embodiments of the present invention provide a hyperspectral target tracking method based on joint spectral dimensionality reduction and feature fusion, such as figure 1 The method shown is:
[0076] Step 1: Load the first frame image of the hyperspectral image sequence, and preprocess the first frame image of the hyperspectral image sequence;
[0077] Specific steps are as follows:
[0078] Step 101, read in the first frame image of the hyperspectral image sequence;
[0079] Specifically, the hyperspectral image sequence in the embodiment of the present invention has 16 channels, so the image size of the first frame read in is M×N×16, where M×N is the scene size.
[0080] Step 102, use a rectangular frame to frame the target image area to be tracked in the first frame image of the hyperspectral image sequence, and use the target image area to be tracked as the...
PUM
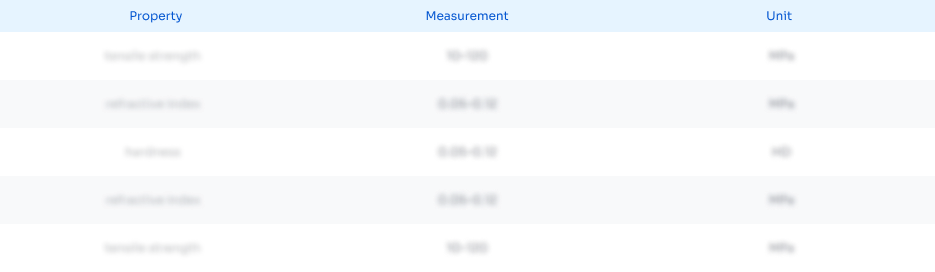
Abstract
Description
Claims
Application Information
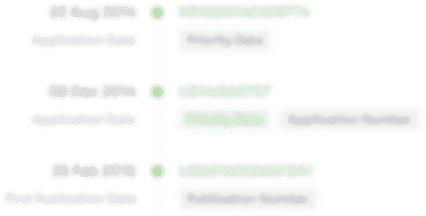
- R&D Engineer
- R&D Manager
- IP Professional
- Industry Leading Data Capabilities
- Powerful AI technology
- Patent DNA Extraction
Browse by: Latest US Patents, China's latest patents, Technical Efficacy Thesaurus, Application Domain, Technology Topic, Popular Technical Reports.
© 2024 PatSnap. All rights reserved.Legal|Privacy policy|Modern Slavery Act Transparency Statement|Sitemap|About US| Contact US: help@patsnap.com