A Multi-Task Detection Method for Pixel-Level Segmentation of Surface Anomaly Regions
A technology of abnormal detection and abnormal area, applied in image analysis, image data processing, instruments, etc., can solve the problems of poor feature extraction, poor effect, poor real-time performance, etc., to improve segmentation fineness and real-time performance, Solve the difficulty of multi-scale information extraction and solve the effect of poor discrimination ability
- Summary
- Abstract
- Description
- Claims
- Application Information
AI Technical Summary
Problems solved by technology
Method used
Image
Examples
Embodiment Construction
[0042] The technical solutions in the embodiments of the present invention will be clearly and completely described below with reference to the accompanying drawings in the embodiments of the present invention.
[0043] The invention provides a multi-task detection method for pixel-level segmentation of abnormal surface areas, and realizes the pixel-level segmentation of abnormal areas of microfluidic chip images. The microfluidic chip is a micro-nanostructure chip commonly used in biochemical experiments, such as figure 1 shown, including the following steps:
[0044]Step 1: Acquire the image data of the microfluidic chip, and select the acquired anomalies for pixel-level annotation. If anomalous samples cannot be obtained, an anomaly mask is generated by randomly specifying initial points, randomly generating an area, and then adding noise conforming to a Gaussian distribution to each pixel within the range of the anomaly mask.
[0045] Each chip image was normalized to 55...
PUM
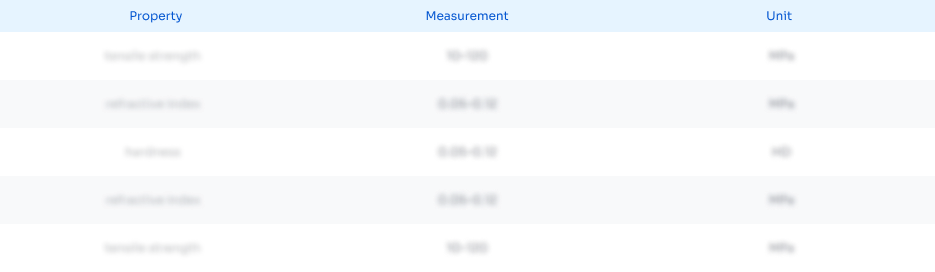
Abstract
Description
Claims
Application Information
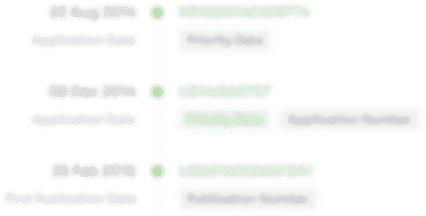
- R&D Engineer
- R&D Manager
- IP Professional
- Industry Leading Data Capabilities
- Powerful AI technology
- Patent DNA Extraction
Browse by: Latest US Patents, China's latest patents, Technical Efficacy Thesaurus, Application Domain, Technology Topic, Popular Technical Reports.
© 2024 PatSnap. All rights reserved.Legal|Privacy policy|Modern Slavery Act Transparency Statement|Sitemap|About US| Contact US: help@patsnap.com