Liver tumor segmentation method and system based on deep learning
A liver tumor, deep learning technology, applied in the field of medical image processing and application, can solve the problems of timeliness, generality and poor accuracy
- Summary
- Abstract
- Description
- Claims
- Application Information
AI Technical Summary
Problems solved by technology
Method used
Image
Examples
Embodiment 1
[0023] Such as figure 1 and figure 2 As shown, this embodiment provides a liver tumor segmentation method based on deep learning, including the following steps: Step S1: Preprocessing the collected data set; Step S2: Building a network model based on the preprocessed data, wherein the The network model includes a plurality of lower convolutional layers and a plurality of upper convolutional layers, the lower convolutional layer is connected to the upper convolutional layer by skipping, and the features obtained by the lower convolutional layer are used in the skipping connection The graph is connected to the upper convolution through the attention module; step S3: input the preprocessed data into the network model for training to obtain the best network model; step S4: use the best network model to treat The processed CT images are segmented to obtain liver tumor regions.
[0024] In the liver tumor segmentation method based on deep learning described in this embodiment, in...
Embodiment 2
[0068] Based on the same inventive concept, this embodiment provides a liver tumor segmentation system based on deep learning, and its problem-solving principle is similar to that of the liver tumor segmentation method based on deep learning, and the repetition will not be repeated.
[0069] This embodiment provides a liver tumor segmentation system based on deep learning, including:
[0070] The preprocessing module is used to preprocess the collected data sets;
[0071] A building module for building a network model based on preprocessed data, wherein the network model includes a plurality of lower convolutional layers and a plurality of upper convolutional layers, and the lower convolutional layers are connected to the upper convolutional layers by skipping Layer, and the feature map obtained by the lower convolution layer is connected to the upper convolution through the attention module on the skip connection;
[0072] A training module, configured to input preprocessed ...
PUM
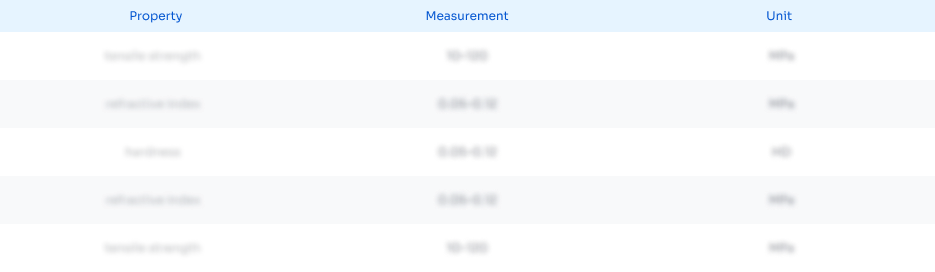
Abstract
Description
Claims
Application Information
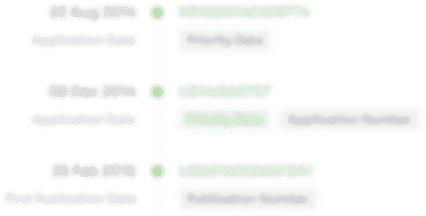
- R&D Engineer
- R&D Manager
- IP Professional
- Industry Leading Data Capabilities
- Powerful AI technology
- Patent DNA Extraction
Browse by: Latest US Patents, China's latest patents, Technical Efficacy Thesaurus, Application Domain, Technology Topic, Popular Technical Reports.
© 2024 PatSnap. All rights reserved.Legal|Privacy policy|Modern Slavery Act Transparency Statement|Sitemap|About US| Contact US: help@patsnap.com