Video behavior recognition method and system based on channel attention-oriented time modeling
A recognition method and attention technology, applied in character and pattern recognition, neural learning methods, biological neural network models, etc., can solve problems such as heavy computational burden and large amount of computation.
- Summary
- Abstract
- Description
- Claims
- Application Information
AI Technical Summary
Problems solved by technology
Method used
Image
Examples
Embodiment 1
[0054] In one or more embodiments, a video behavior recognition method based on channel attention-oriented temporal modeling is disclosed, referring to figure 1 , including the following procedures:
[0055] (1) Obtain the convolution feature map of the input behavior video;
[0056] (2) Generate channel attention weights and adjust the input video convolution feature map;
[0057] (3) Select the feature channel whose attention weight is higher than the set value to perform residual time modeling, and calculate the residual of the spatial features of adjacent frames in these channels to establish a temporal correlation model between them, by capturing the human body The motion dynamics of actions changing over time to learn the temporal relationship of the video, and then obtain a more discriminative video feature representation;
[0058] (4) Perform video behavior recognition based on the obtained feature representation.
[0059] Specifically, given a convolutional feature...
Embodiment 2
[0136] In one or more embodiments, a video behavior recognition system based on channel attention-oriented temporal modeling is disclosed, characterized in that it includes:
[0137] The data acquisition module is used to obtain the convolution feature map of the input behavior video;
[0138] Channel attention generation (CAG) module, used to obtain channel weights and adjust the original input video convolution feature map;
[0139] The Residual Time Modeling (RTM) module is used to select the feature channels whose attention weight is higher than the set value for residual time modeling, and calculate the residuals of the spatial features of adjacent frames in these channels to establish the relationship between them. The temporal correlation model of the human body learns the temporal relationship of the video by capturing the dynamics of human motion over time, and then obtains a more discriminative video feature representation;
[0140] The video behavior recognition mo...
Embodiment 3
[0146] In one or more embodiments, a terminal device is disclosed, including a server, the server includes a memory, a processor, and a computer program stored on the memory and operable on the processor, and the processor executes the The program implements the video behavior recognition method based on channel attention-oriented temporal modeling in the first embodiment. For the sake of brevity, details are not repeated here.
PUM
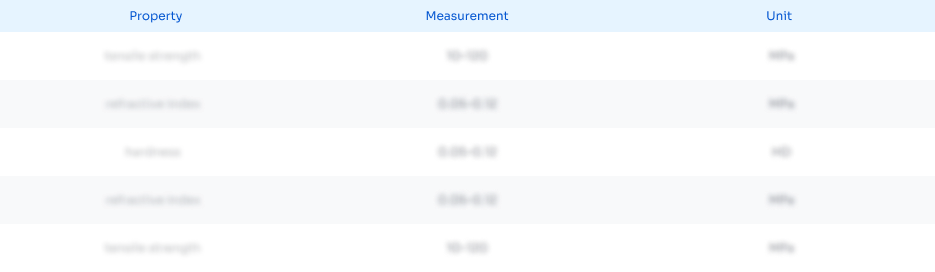
Abstract
Description
Claims
Application Information
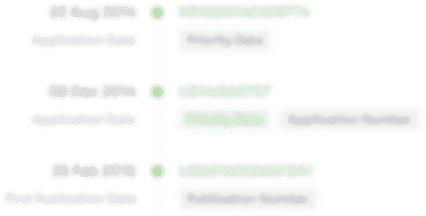
- R&D Engineer
- R&D Manager
- IP Professional
- Industry Leading Data Capabilities
- Powerful AI technology
- Patent DNA Extraction
Browse by: Latest US Patents, China's latest patents, Technical Efficacy Thesaurus, Application Domain, Technology Topic, Popular Technical Reports.
© 2024 PatSnap. All rights reserved.Legal|Privacy policy|Modern Slavery Act Transparency Statement|Sitemap|About US| Contact US: help@patsnap.com