Federal learning method, storage medium, terminal, server and federal learning system
A learning method and storage medium technology, applied in integrated learning and other directions, can solve problems such as inability to effectively take into account terminal power consumption and overall training time, inability to analyze data on mobile terminals, and violation of data analysis, so as to reduce overall training time and reduce training. time, and the effect of improving training efficiency
- Summary
- Abstract
- Description
- Claims
- Application Information
AI Technical Summary
Problems solved by technology
Method used
Image
Examples
Embodiment 1
[0066] Such as figure 1 As shown, the federated learning system according to Embodiment 1 of the present invention includes a server 10 and several terminals 20, and the server 10 and the terminals 20 can communicate with each other. The basic workflow of the federated learning system is: each terminal 20 sends its own training information to the server 10, the server 10 obtains the constraint time according to the training information of each terminal 20, the terminal 20 obtains the training parameters according to the constraint time, and the terminal 20 obtains the training parameters according to the specified time limit. The above training parameters are used to complete the model training. The constraint time is used to enable the terminal to complete the model training within the constraint time, and the training parameters are used to enable the terminal to consume the minimum energy consumption when completing the model training. In this way, the federated learning s...
Embodiment 2
[0069] Such as figure 2 As shown, the federated learning method in the second embodiment includes the following steps:
[0070] Step S10: the server 10 receives the training information sent from the terminal.
[0071] Before starting training each time, the server 10 needs to collect training information of each terminal 20 in advance. The training information includes hardware information and a preset amount of training data, wherein the hardware information includes information such as the processor information of the terminal, mainly refers to information such as CPU frequency and CPU cycle, and the preset amount of training data refers to information such as Contains the number of training data, for example, for image recognition tasks, the number of training data refers to how many pictures are contained in the terminal.
[0072] Step S20: the server 10 acquires a constraint time according to the training information, and the constraint time is used to enable the term...
Embodiment 3
[0097] Embodiment 3 discloses a computer-readable storage medium, where a federated learning program is stored in the computer-readable storage medium, and when the federated learning program is executed by a processor, the federated learning method as described in Embodiment 2 is implemented.
PUM
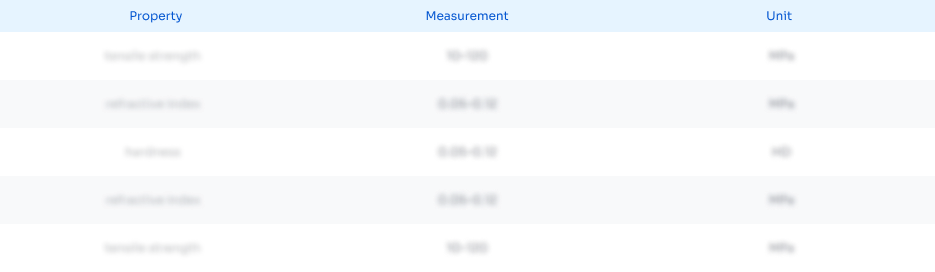
Abstract
Description
Claims
Application Information
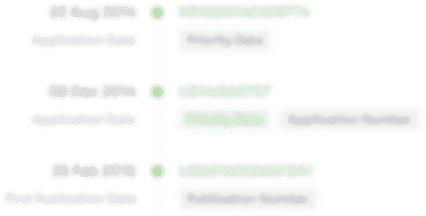
- Generate Ideas
- Intellectual Property
- Life Sciences
- Materials
- Tech Scout
- Unparalleled Data Quality
- Higher Quality Content
- 60% Fewer Hallucinations
Browse by: Latest US Patents, China's latest patents, Technical Efficacy Thesaurus, Application Domain, Technology Topic, Popular Technical Reports.
© 2025 PatSnap. All rights reserved.Legal|Privacy policy|Modern Slavery Act Transparency Statement|Sitemap|About US| Contact US: help@patsnap.com