Modulation mode recognition method based on spatial-temporal feature extraction deep learning
A technology of spatiotemporal features and modulation patterns, applied in modulation type recognition, neural learning methods, character and pattern recognition, etc., can solve problems such as online processing, high complexity of recognition models, high model parameter quantity and complexity
- Summary
- Abstract
- Description
- Claims
- Application Information
AI Technical Summary
Problems solved by technology
Method used
Image
Examples
Embodiment Construction
[0050] In order to make the object, technical solution and advantages of the present invention clearer, the present invention will be further described in detail below in conjunction with the accompanying drawings and embodiments. It should be understood that the specific embodiments described here are only used to explain the present invention, not to limit the present invention.
[0051] Please refer to figure 1 , the present invention provides a modulation pattern recognition method based on spatio-temporal feature extraction deep learning, the method comprising the following steps:
[0052] S1. Collect signals of modulation modes to be identified;
[0053] S2, please refer to figure 2 (Where Input is the input layer; I, Q represent I and Q respectively; Dense is the fully connected layer; Activation is the activation function layer; Dropout is the layer to prevent the model from overfitting; Concatenate is the data splicing layer; Conv2d is the convolution layer; GRU i...
PUM
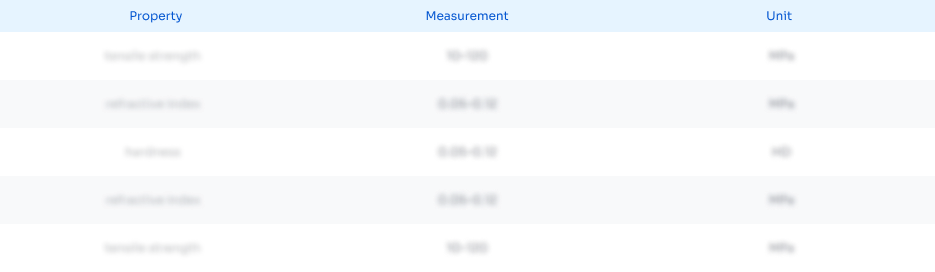
Abstract
Description
Claims
Application Information
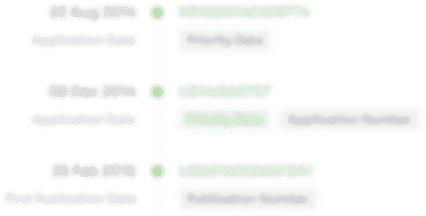
- R&D
- Intellectual Property
- Life Sciences
- Materials
- Tech Scout
- Unparalleled Data Quality
- Higher Quality Content
- 60% Fewer Hallucinations
Browse by: Latest US Patents, China's latest patents, Technical Efficacy Thesaurus, Application Domain, Technology Topic, Popular Technical Reports.
© 2025 PatSnap. All rights reserved.Legal|Privacy policy|Modern Slavery Act Transparency Statement|Sitemap|About US| Contact US: help@patsnap.com