A third-party federated gradient boosting decision tree model training method
A decision tree and gradient technology, applied in character and pattern recognition, data processing applications, finance, etc., can solve problems such as data leakage, space consumption, and difficulty in finding a trusted third party, so as to protect data security and reduce storage space , to ensure the effect of training accuracy
- Summary
- Abstract
- Description
- Claims
- Application Information
AI Technical Summary
Problems solved by technology
Method used
Image
Examples
Embodiment
[0100] Embodiment: A third-party-free federated gradient boosting decision tree model training method in this embodiment is used for joint risk control modeling between banks and operators, such as figure 1 shown, including the following steps:
[0101] S1: The training initiator and training participants synchronously initialize the model parameters of their respective federated gradient boosting decision tree models; the model parameters include the depth of the federated gradient boosting decision tree, the number of federated gradient boosting decision trees, the sampling rate of large gradient samples, and the small gradient Sample sampling rate, tree column sampling rate, tree row sampling rate, learning rate, maximum number of leaves, minimum number of node samples after splitting, minimum profit of splitting, number of bins, L2 regularization, L1 regularization, termination threshold, modeling method;
[0102] S2: The training initiator samples d sample data sets x fro...
PUM
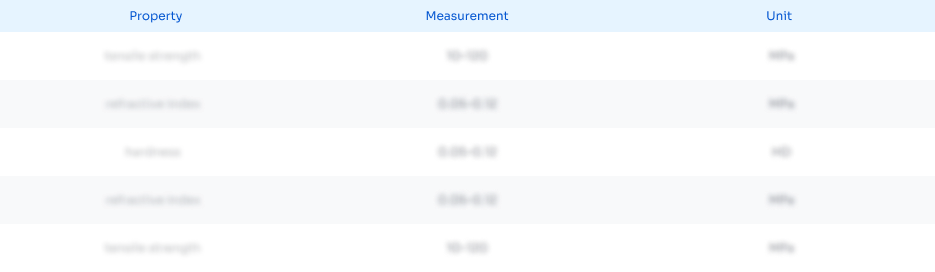
Abstract
Description
Claims
Application Information
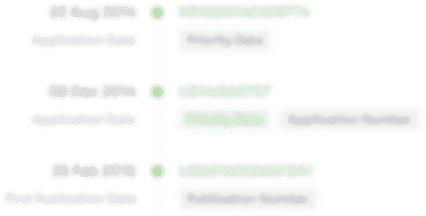
- R&D Engineer
- R&D Manager
- IP Professional
- Industry Leading Data Capabilities
- Powerful AI technology
- Patent DNA Extraction
Browse by: Latest US Patents, China's latest patents, Technical Efficacy Thesaurus, Application Domain, Technology Topic, Popular Technical Reports.
© 2024 PatSnap. All rights reserved.Legal|Privacy policy|Modern Slavery Act Transparency Statement|Sitemap|About US| Contact US: help@patsnap.com