3D shape image classification method of isovariant 3D convolutional network based on partial differential operator
A technology of convolutional network and classification method, which is applied in the field of 3D shape classification, can solve the problems that discrete groups cannot be included, cannot be used to deal with discrete groups, and commonly used groups and group representations cannot be covered in one unity, achieving low 3D Shape classification error rate, effect of improving parameter utilization
- Summary
- Abstract
- Description
- Claims
- Application Information
AI Technical Summary
Problems solved by technology
Method used
Image
Examples
Embodiment Construction
[0054] Below in conjunction with accompanying drawing, further describe the present invention through embodiment, but do not limit the scope of the present invention in any way.
[0055] The present invention provides a 3D shape classification method based on the partial differential operator-based equivariant 3D convolutional network model PDO-e3DCNN. The partial differential operator is used to design an equivariant 3D convolutional network model for efficient 3D shape classification. Visual analysis such as classification and recognition.
[0056] image 3 Shown is the specific implementation of the present invention to realize the method flow of 3D shape classification based on the equivariant 3D convolution network model of partial differential operator, including the following steps:
[0057] Step 1: Divide 3D shapes into training samples and test samples. All data sets in this example are rotated SHREC'17 data sets, which consist of 51,162 3D shapes, of which 35,764 ar...
PUM
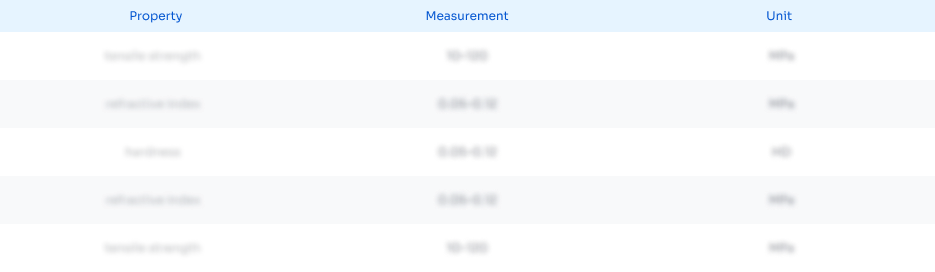
Abstract
Description
Claims
Application Information
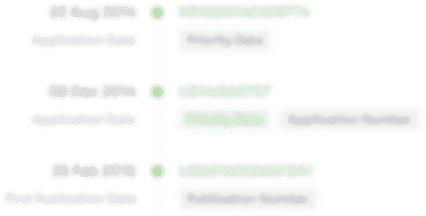
- R&D
- Intellectual Property
- Life Sciences
- Materials
- Tech Scout
- Unparalleled Data Quality
- Higher Quality Content
- 60% Fewer Hallucinations
Browse by: Latest US Patents, China's latest patents, Technical Efficacy Thesaurus, Application Domain, Technology Topic, Popular Technical Reports.
© 2025 PatSnap. All rights reserved.Legal|Privacy policy|Modern Slavery Act Transparency Statement|Sitemap|About US| Contact US: help@patsnap.com