Design method of image classification loss function based on cosine space optimization
A technology of loss function and design method, applied in the field of computer vision and artificial intelligence, can solve problems such as large parameter quantity and computational complexity, and achieve the effect of improving model performance
- Summary
- Abstract
- Description
- Claims
- Application Information
AI Technical Summary
Problems solved by technology
Method used
Image
Examples
Embodiment 1
[0037] This implementation provides an image classification loss function based on cosine space optimization. The loss function consists of three parts, and the specific expression is as follows:
[0038] Loss = αLoss 1 +β·Truc(Loss 2 -ε)+γLoss cross-entropy
[0039] In the formula, α is the weighting coefficient of the inter-class loss function, β is the weighting coefficient of the intra-class loss function, and γ is the weighting coefficient of the cross-entropy loss function, and the ratio of γ>α>β is adopted; Loss 1 Expressed as a between-class loss function, Loss cross-entropy Expressed as a cross-entropy loss function, Loss 2 Expressed as an intra-class loss function;
[0040] The hyperparameter β is a piecewise function. When the batch of algorithm model training is smaller than the hyperparameter N, β takes a value of 0, and when the training batch exceeds N, then β takes a value greater than 0.
[0041] The value of the hyperparameter N is determined according ...
Embodiment 2
[0055] This embodiment provides a method for designing an image classification loss function based on cosine space optimization, including the following steps:
[0056] Step S1, obtaining a data set, setting hyperparameters, and initializing a deep learning model;
[0057] Specifically, in this embodiment, the above hyperparameters include: weighting coefficient α, weighting coefficient β, weighting coefficient γ, compaction coefficient ε, and batch number N of iterative training, and satisfy: γ>α>β.
[0058] Step S2, input the acquired data set into the initialized deep learning model, perform multi-batch iterative training on the deep learning model, and execute step S21-step S23 sequentially in each iterative batch;
[0059] Step S21, according to the feature vector obtained by the deep learning model in the forward propagation process, calculate the intra-class center c of each category object in the current iteration batch i , and cumulatively update the in-class center ...
PUM
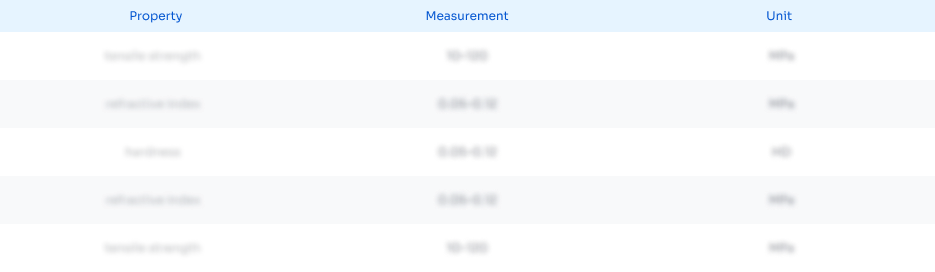
Abstract
Description
Claims
Application Information
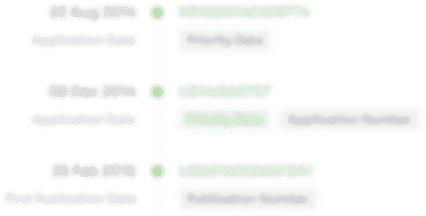
- R&D Engineer
- R&D Manager
- IP Professional
- Industry Leading Data Capabilities
- Powerful AI technology
- Patent DNA Extraction
Browse by: Latest US Patents, China's latest patents, Technical Efficacy Thesaurus, Application Domain, Technology Topic, Popular Technical Reports.
© 2024 PatSnap. All rights reserved.Legal|Privacy policy|Modern Slavery Act Transparency Statement|Sitemap|About US| Contact US: help@patsnap.com