Traffic signal lamp scheduling method and system based on iterative learning model predictive control
A technology of model predictive control and traffic lights, which is applied in traffic signal control, road vehicle traffic control system, traffic control system, etc., can solve the problems of inability to respond to random changes in traffic demand, and the inability to guarantee terminal set convexity, etc. To achieve the effect of optimizing performance
- Summary
- Abstract
- Description
- Claims
- Application Information
AI Technical Summary
Problems solved by technology
Method used
Image
Examples
Embodiment Construction
[0063] The following describes the processes executed by multiple preferred algorithms of the present invention with reference to the accompanying drawings, so as to make the technical content clearer and easier to understand. The present invention can be embodied by many different forms of algorithms, and the protection scope of the present invention is not limited to the algorithms mentioned in the text.
[0064] Such as figure 1 As shown, it is a working flow chart of a traffic signal dispatching method based on iterative learning model predictive control provided by the present invention. Specifically, the method includes the following steps:
[0065] Step 1. Establish a traffic flow simulation model in the target area;
[0066] Step 2. Obtain closed-loop data of a fixed time interval according to the control strategy;
[0067] Step 3, using the closed-loop data as the terminal condition of the subsequent open-loop predictive optimization problem to perform predictive c...
PUM
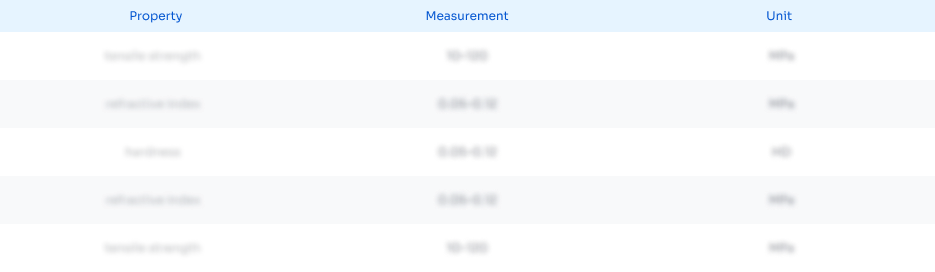
Abstract
Description
Claims
Application Information
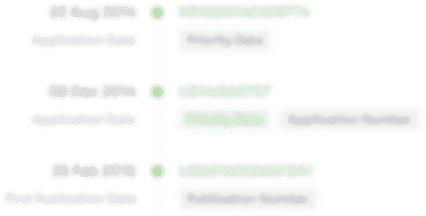
- R&D Engineer
- R&D Manager
- IP Professional
- Industry Leading Data Capabilities
- Powerful AI technology
- Patent DNA Extraction
Browse by: Latest US Patents, China's latest patents, Technical Efficacy Thesaurus, Application Domain, Technology Topic.
© 2024 PatSnap. All rights reserved.Legal|Privacy policy|Modern Slavery Act Transparency Statement|Sitemap