Convolutional neural network-extremely randomized trees (CNN-ET) model-based electrocardiogram monitoring method
A technology of electrical monitoring and modeling, applied in diagnostic recording/measurement, medical science, sensors, etc., can solve problems such as inability to effectively and accurately realize ECG signal classification and recognition, and low accuracy of classification models
- Summary
- Abstract
- Description
- Claims
- Application Information
AI Technical Summary
Problems solved by technology
Method used
Image
Examples
Embodiment
[0047] Embodiment: a kind of ECG monitoring method based on CNN-ET model of the present embodiment, such as figure 1 shown, including the following steps:
[0048] S1. Collect the ECG signal of the subject to construct the original data set, and obtain the training data and test data. The ECG signal of the subject is as follows: figure 2 shown;
[0049]S2. Normalize the training data and test data to obtain training samples and test samples;
[0050] S3, building a CNN-EF hybrid model;
[0051] S4. Input the training samples into the CNN-ET hybrid model for training to obtain a trained CNN-ET hybrid model;
[0052] S5. Input the test sample into the trained CNN-ET hybrid model to classify and recognize the ECG signal.
[0053] The CNN-ET model organically combines convolutional neural network (CNN) and extreme random number (ET), integrates the feature extraction of ECG signals and the classification tasks of ECG signals, and improves the relevance and classification accu...
PUM
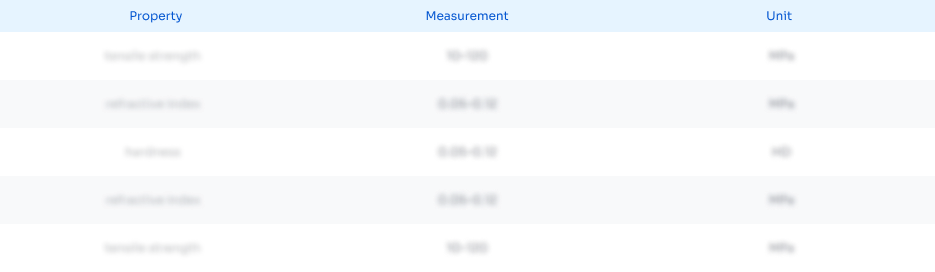
Abstract
Description
Claims
Application Information
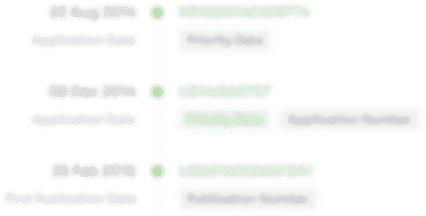
- R&D Engineer
- R&D Manager
- IP Professional
- Industry Leading Data Capabilities
- Powerful AI technology
- Patent DNA Extraction
Browse by: Latest US Patents, China's latest patents, Technical Efficacy Thesaurus, Application Domain, Technology Topic, Popular Technical Reports.
© 2024 PatSnap. All rights reserved.Legal|Privacy policy|Modern Slavery Act Transparency Statement|Sitemap|About US| Contact US: help@patsnap.com