Initial fault identification method for active power distribution system based on time-frequency memory recurrent neural network
A recurrent neural network and initial failure technology, applied in neural learning methods, biological neural network models, neural architectures, etc., can solve related problems, application problems, inappropriate detection equipment initial failures and other problems, achieve strong generalization, The effect of meeting identification requirements and high-precision fault identification
- Summary
- Abstract
- Description
- Claims
- Application Information
AI Technical Summary
Problems solved by technology
Method used
Image
Examples
Embodiment Construction
[0055] In this embodiment, a method for identifying initial faults in an active power distribution system based on a time-frequency memory recursive neural network is performed in the following steps:
[0056] Step 1, collecting three-phase current and three-phase voltage data;
[0057] Step 2, preprocessing the collected three-phase current and three-phase voltage data, including data enhancement and normalization processing;
[0058] The collected three-phase current data is I a , I b , I c , the three-phase voltage data is V a , V b , V c , rotate the three-phase data to get [I a I b I c V a V b V c ], [I a I c I b V a V c V b ], [I b I a I c V b V a V c ],
[0059] [I b I c I a V b V c V a ], [I c I b I a V c V b V a ], [I c I a I b V c V a V b ] Six groups of data; in the present embodiment, by adding a time window with a length of 164, the translation step is 10 to carry out time series expansion to ...
PUM
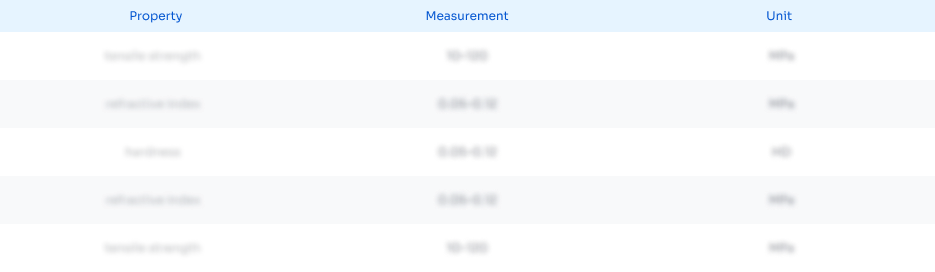
Abstract
Description
Claims
Application Information
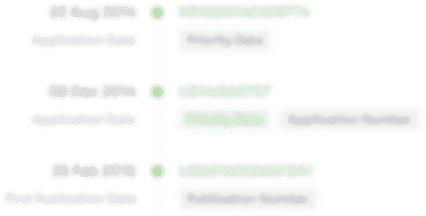
- R&D Engineer
- R&D Manager
- IP Professional
- Industry Leading Data Capabilities
- Powerful AI technology
- Patent DNA Extraction
Browse by: Latest US Patents, China's latest patents, Technical Efficacy Thesaurus, Application Domain, Technology Topic, Popular Technical Reports.
© 2024 PatSnap. All rights reserved.Legal|Privacy policy|Modern Slavery Act Transparency Statement|Sitemap|About US| Contact US: help@patsnap.com