Knowledge graph joint representation learning method fusing graph convolution and translation model
- Summary
- Abstract
- Description
- Claims
- Application Information
AI Technical Summary
Problems solved by technology
Method used
Image
Examples
Embodiment Construction
[0053] The present invention will be further described below in conjunction with accompanying drawing.
[0054] refer to figure 1 and figure 2 , a knowledge graph joint representation learning method that integrates graph convolution and translation models, including the following steps:
[0055] 1) Construct knowledge map adjacency matrix
[0056] For knowledge graphs that need to obtain their embedded representations through representation learning, their adjacency matrix is constructed according to the connection relationship between entities to figure 1 Take the knowledge graph as an example, figure 1 (a) is a knowledge graph constructed based on David Beckham's interpersonal relationship data, which is abstracted as figure 1 the representation of (b);
[0057] The direct adjacency matrix reflects whether entities are directly connected, for example, figure 1 Entity e 1 with e 2 connected then a 12 =1, a∈A, therefore, figure 1 The direct adjacency matrix A of ...
PUM
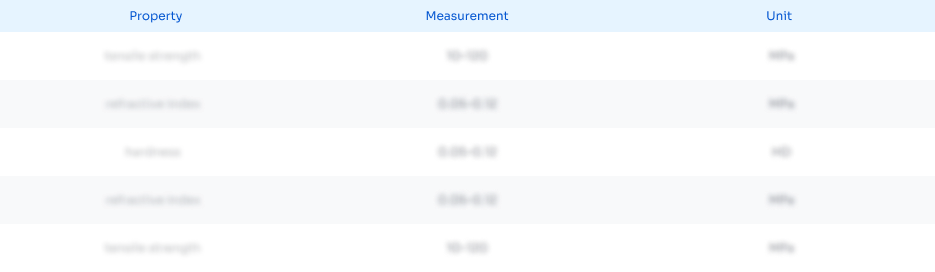
Abstract
Description
Claims
Application Information
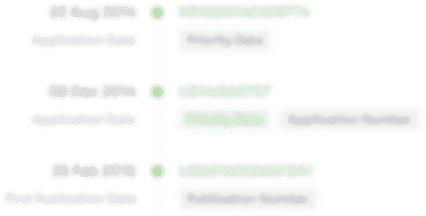
- R&D
- Intellectual Property
- Life Sciences
- Materials
- Tech Scout
- Unparalleled Data Quality
- Higher Quality Content
- 60% Fewer Hallucinations
Browse by: Latest US Patents, China's latest patents, Technical Efficacy Thesaurus, Application Domain, Technology Topic, Popular Technical Reports.
© 2025 PatSnap. All rights reserved.Legal|Privacy policy|Modern Slavery Act Transparency Statement|Sitemap|About US| Contact US: help@patsnap.com