A Method for Learning Labeled Noisy Images Based on Dual Active Query
An active, image technology, applied in the field of image learning, can solve the problem of noise label noise transfer matrix estimation bias, and achieve the effect of avoiding estimation bias
- Summary
- Abstract
- Description
- Claims
- Application Information
AI Technical Summary
Problems solved by technology
Method used
Image
Examples
Embodiment Construction
[0025] This embodiment describes a method for learning tagged images with noise based on dual active query.
[0026] Such as figure 1 As shown, the method includes the following steps:
[0027] Step 1. Get image set X and noise label set Y , forming a noisy data set D =(X, Y ).
[0028] The labels of the image set X can be obtained by manual labeling, such as asking experts or crowdsourcing, etc.; they can also be obtained by automatic collection, such as crawlers. However, the marks actually collected by these methods Y contains a large number of wrong labels.
[0029] If a deep neural network classifier model is directly learned on these real data sets, it will overfit the noisy data, resulting in a decrease in its own generalization performance. Existing learning theories suggest that:
[0030] The noise is modeled by the noise transfer matrix. When the noise transfer matrix is estimated accurately, the optimal classifier on the noise data is equivalent to the opt...
PUM
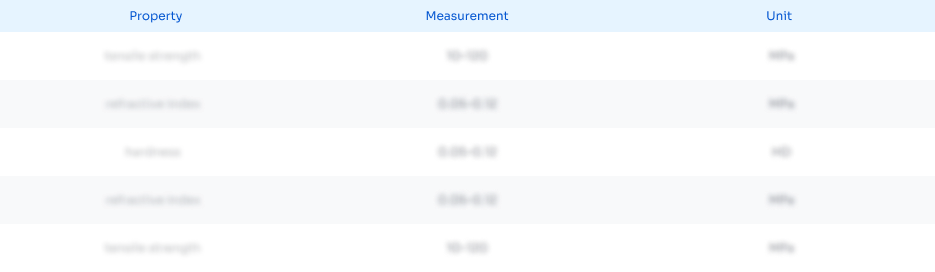
Abstract
Description
Claims
Application Information
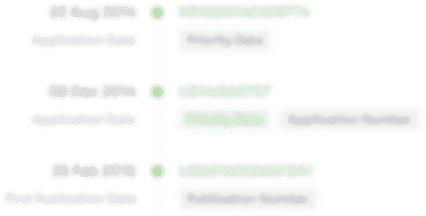
- R&D Engineer
- R&D Manager
- IP Professional
- Industry Leading Data Capabilities
- Powerful AI technology
- Patent DNA Extraction
Browse by: Latest US Patents, China's latest patents, Technical Efficacy Thesaurus, Application Domain, Technology Topic.
© 2024 PatSnap. All rights reserved.Legal|Privacy policy|Modern Slavery Act Transparency Statement|Sitemap