A FPC defect detection method based on improved mask RCNN
A defect detection and defect technology, applied in neural learning methods, image enhancement, instruments, etc., to achieve the effect of saving labor costs and facilitating re-judgment
- Summary
- Abstract
- Description
- Claims
- Application Information
AI Technical Summary
Problems solved by technology
Method used
Image
Examples
Embodiment
[0045] In this embodiment, based on the improved MASK RCNN FPC defect detection method, firstly, the collected original FPC image is pre-processed, and then the pre-processed image is data enhanced to expand the amount of FPC image data, and then the enhanced image The data is manually marked, and the marked data set is divided into a training set and a verification set according to a ratio of 4:1, and then the divided data set is sent to the model training, and finally the trained model is evaluated to determine whether the model meets the FPC testing requirements. Specific steps are as follows:
[0046] Step 1: ROI processing and image cropping, collect the target detection image of the industrial site, and preprocess the FPC original image data, including ROI processing and image cropping.
[0047] Step 2: Perform data enhancement processing on the preprocessed image. Data enhancement processing adopts traditional image processing methods: image rotation, image mirroring, ...
PUM
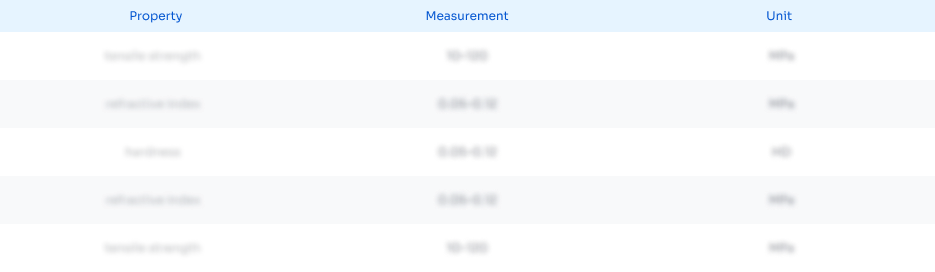
Abstract
Description
Claims
Application Information
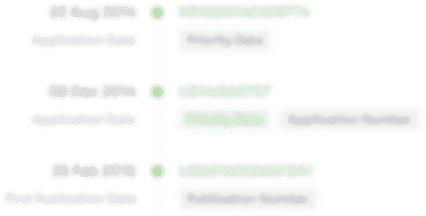
- R&D Engineer
- R&D Manager
- IP Professional
- Industry Leading Data Capabilities
- Powerful AI technology
- Patent DNA Extraction
Browse by: Latest US Patents, China's latest patents, Technical Efficacy Thesaurus, Application Domain, Technology Topic, Popular Technical Reports.
© 2024 PatSnap. All rights reserved.Legal|Privacy policy|Modern Slavery Act Transparency Statement|Sitemap|About US| Contact US: help@patsnap.com