Electric locomotive idling online identification method based on fuzzy entropy and kernel extreme learning machine
A nuclear extreme learning machine and extreme learning machine technology, applied in the field of rail transit train traction control, can solve the problems of not effectively extracting high-precision features of signals, online recognition accuracy, rapidity, online linearity or anti-interference defects, etc.
- Summary
- Abstract
- Description
- Claims
- Application Information
AI Technical Summary
Problems solved by technology
Method used
Image
Examples
Embodiment Construction
[0044] An online identification method for electric locomotive idling based on multi-scale fuzzy entropy (MSFE) and kernel extreme learning machine (KELM), the method flow chart is as follows Image 6 As shown, where the input is the locomotive wheel set speed v(t), and the output is the identified idling state. The technical features involved in this method mainly include three main modules: multi-scale fuzzy entropy feature extraction, optimal kernel extreme learning machine model, and idling online recognition.
[0045] Among them, the main function of the multi-scale fuzzy entropy feature extraction module is to calculate the fuzzy entropy value on the τ scale for the locomotive wheel set speed v(t), and then extract the feature to obtain the τ-dimensional feature vector that can represent the sticking / idling state of the locomotive, and iteratively calculate The feature vector at each time point is then constructed into a feature matrix output; the optimal kernel extreme ...
PUM
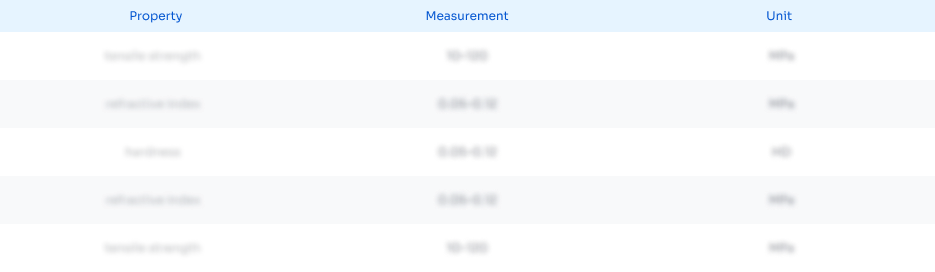
Abstract
Description
Claims
Application Information
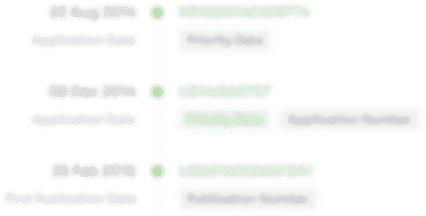
- R&D Engineer
- R&D Manager
- IP Professional
- Industry Leading Data Capabilities
- Powerful AI technology
- Patent DNA Extraction
Browse by: Latest US Patents, China's latest patents, Technical Efficacy Thesaurus, Application Domain, Technology Topic.
© 2024 PatSnap. All rights reserved.Legal|Privacy policy|Modern Slavery Act Transparency Statement|Sitemap