Power distribution network investment decision-making method based on deep transfer learning
A technology of transfer learning and decision-making method, which is applied in the field of distribution network investment decision-making based on deep transfer learning, and can solve problems such as lack of power grid data sample support, complex and diverse distribution network upgrading and transformation measures, and benefit gap
- Summary
- Abstract
- Description
- Claims
- Application Information
AI Technical Summary
Problems solved by technology
Method used
Image
Examples
Embodiment
[0025] Such as Figure 1 to Figure 2 As shown, the distribution network investment decision-making method based on deep transfer learning mainly includes three parts: data collection and screening, edge distribution adaptation, and conditional distribution adaptation. The specific process of each part is as follows:
[0026] S10. Data collection and screening: Since power grid investment planning usually takes years as a cycle, the historical operation data of the target distribution network is often difficult to meet the sample size requirements required for deep learning training, so collecting n R The historical data of a distribution network is used as the original data set D for migration learning of the target distribution network R ={d R (1),d R (2),...,d R (n R )}.
[0027] Based on the maximum mean difference MMD to measure the difference between variables in different data sets, its mathematical expression is:
[0028]
[0029] in, Represents the source do...
PUM
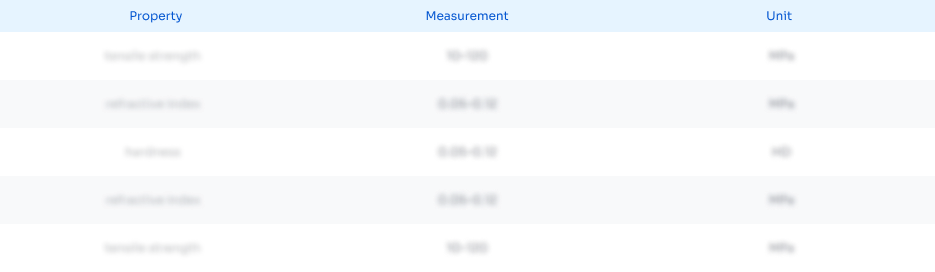
Abstract
Description
Claims
Application Information
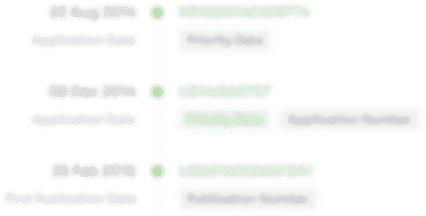
- R&D
- Intellectual Property
- Life Sciences
- Materials
- Tech Scout
- Unparalleled Data Quality
- Higher Quality Content
- 60% Fewer Hallucinations
Browse by: Latest US Patents, China's latest patents, Technical Efficacy Thesaurus, Application Domain, Technology Topic, Popular Technical Reports.
© 2025 PatSnap. All rights reserved.Legal|Privacy policy|Modern Slavery Act Transparency Statement|Sitemap|About US| Contact US: help@patsnap.com