Reinforcement learning model optimization method and device, storage medium and electronic equipment
An optimization method and reinforcement learning technology, applied in the field of deep reinforcement learning, can solve problems such as lack of generalization ability, difficulty in reproducing, and insufficient utilization of experience, and achieve the goals of improving learning quality, good usability, and good generalization Effect
- Summary
- Abstract
- Description
- Claims
- Application Information
AI Technical Summary
Problems solved by technology
Method used
Image
Examples
Embodiment Construction
[0026] The following will clearly and completely describe the technical solutions in the embodiments of the application with reference to the drawings in the embodiments of the application. Apparently, the described embodiments are only some of the embodiments of the application, not all of them. Based on the embodiments in this application, all other embodiments obtained by persons of ordinary skill in the art without making creative efforts belong to the scope of protection of this application.
[0027] In the description of the present application, it should be understood that the terms "first", "second" and so on are used for descriptive purposes only, and should not be understood as indicating or implying relative importance. In the description of the present application, it should be noted that, unless otherwise specified and limited, "including" and "having" and any variations thereof are intended to cover non-exclusive inclusion. For example, a process, method, system,...
PUM
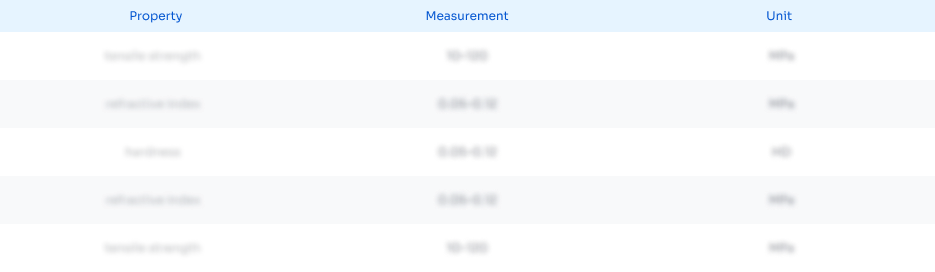
Abstract
Description
Claims
Application Information
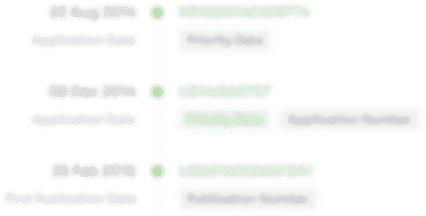
- R&D
- Intellectual Property
- Life Sciences
- Materials
- Tech Scout
- Unparalleled Data Quality
- Higher Quality Content
- 60% Fewer Hallucinations
Browse by: Latest US Patents, China's latest patents, Technical Efficacy Thesaurus, Application Domain, Technology Topic, Popular Technical Reports.
© 2025 PatSnap. All rights reserved.Legal|Privacy policy|Modern Slavery Act Transparency Statement|Sitemap|About US| Contact US: help@patsnap.com