Cross-view gait recognition method based on subspace learning of joint hierarchical selection
A technology of subspace learning and gait recognition, applied in the field of pattern recognition, can solve problems such as performance degradation, large amount of calculation, and small amount of database data, and achieve the effects of improving performance, enhancing connection, and strong robustness
- Summary
- Abstract
- Description
- Claims
- Application Information
AI Technical Summary
Problems solved by technology
Method used
Image
Examples
Embodiment 1
[0060] A cross-view gait recognition method based on subspace learning of joint hierarchical selection, including:
[0061] 1) First divide the gait samples of the target perspective and the registration perspective into a training set and a test set, wherein the training set includes the gait samples of the target perspective and the gait samples of the registration perspective, and the test set is the gait samples of the target perspective;
[0062] Obtain the gait energy map of the two gait samples of the target view and the registration view, and divide the gait energy map by using a hierarchical block division scheme. The hierarchical block division scheme includes gradually dense grids of different densities. Through different densities The grid generates gait energy map blocks with different sizes, which ensures that the most likely and appropriate ideal blocks that are most relevant to gait information are included. The partition method is as follows image 3 As shown,...
PUM
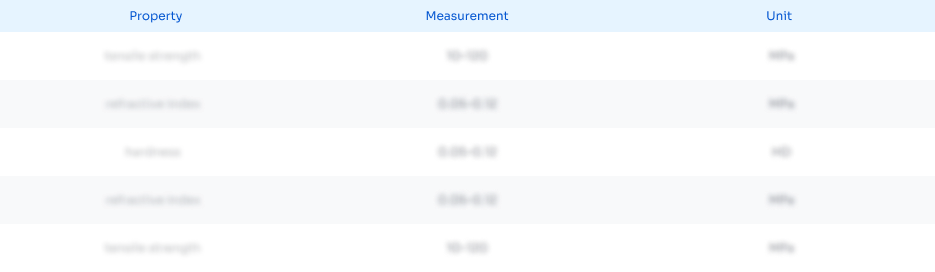
Abstract
Description
Claims
Application Information
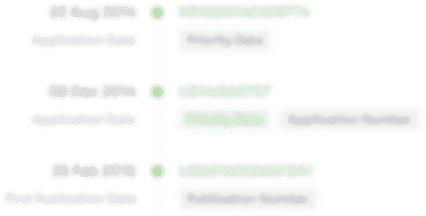
- R&D Engineer
- R&D Manager
- IP Professional
- Industry Leading Data Capabilities
- Powerful AI technology
- Patent DNA Extraction
Browse by: Latest US Patents, China's latest patents, Technical Efficacy Thesaurus, Application Domain, Technology Topic, Popular Technical Reports.
© 2024 PatSnap. All rights reserved.Legal|Privacy policy|Modern Slavery Act Transparency Statement|Sitemap|About US| Contact US: help@patsnap.com