An Incremental Learning Method Based on Diverse Example Sets of Triplets and Gradient Regularization
A triplet and example technology, applied in the field of incremental learning, can solve the problems of not being able to describe the old task optimization space distribution well, not covering the data distribution well, and the direction and size of example data constraint optimization, etc., to achieve Solve catastrophic forgetting, rich variety, good effect
- Summary
- Abstract
- Description
- Claims
- Application Information
AI Technical Summary
Problems solved by technology
Method used
Image
Examples
Embodiment 1
[0101] Embodiment 1. This embodiment will be described with reference to FIG. 1 to FIG. 3. A triplet-based diverse example set of this embodiment will be described.
[0106] where V is the set of features corresponding to a batch of images, φ is the feature extractor, x
[0108]
[0111] S2. After completing the training of the batch data of the first task, input the batch data of the first task into the model again
[0114]
[0117]
[0119] S3. Input the test data into the model, calculate the accuracy of each category, use the accuracy of each category and
[0122] The test data of the c category is input into the model for prediction in turn, and the predicted value of the test sample and the test sample are
[0123]
[0127] Among them, acc represents the accuracy set of all known categories, acc
[0128] S33. Calculate the number of examples that should be stored in the example set of each category; the specific method is:
[0129] Since the example set M is a fixed value, as t...
Embodiment 2
[0188] Experimental analysis of the TROCL model was performed on the image classification dataset cifar-100. The cifar‑100 dataset has
[0189] The categories included in cifar-100 do not have strong inheritance, and there are certain similarities between the sub-categories.
[0190] The present invention will use an online class increment to organize training. Divide 100 classes into 10 tasks, each task
[0191] Image features are extracted using resnet-18. Before entering the model, each image is a 1×3072 tensor. go through
PUM
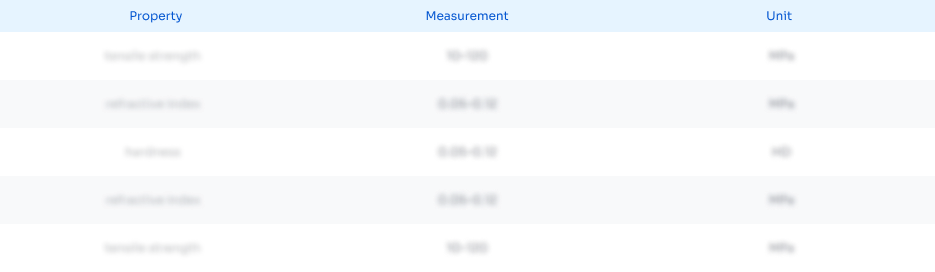
Abstract
Description
Claims
Application Information
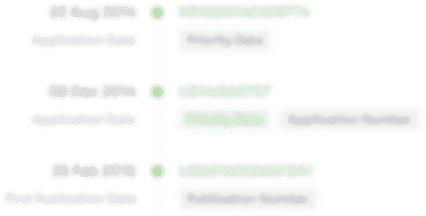
- R&D
- Intellectual Property
- Life Sciences
- Materials
- Tech Scout
- Unparalleled Data Quality
- Higher Quality Content
- 60% Fewer Hallucinations
Browse by: Latest US Patents, China's latest patents, Technical Efficacy Thesaurus, Application Domain, Technology Topic, Popular Technical Reports.
© 2025 PatSnap. All rights reserved.Legal|Privacy policy|Modern Slavery Act Transparency Statement|Sitemap|About US| Contact US: help@patsnap.com