FFCNN-SVM transfer learning fault diagnosis method based on feature fusion under small sample
A technology of transfer learning and feature fusion, applied in neural learning methods, instruments, biological neural network models, etc., can solve problems such as it is difficult to reveal the inherent characteristics of fault samples and not so easy to distinguish, and achieve good fault diagnosis results
- Summary
- Abstract
- Description
- Claims
- Application Information
AI Technical Summary
Problems solved by technology
Method used
Image
Examples
Embodiment Construction
[0012] In order to evaluate the effectiveness of the proposed method, the present invention conducts experiments on the two data sets of the motor rotor data set and the bearing data set.
[0013] Case number one:
[0014] The equipment selected in the motor rotor experiment is the ZHS-2 multifunctional motor test bench with a flexible rotor. A total of 8 sensors are installed in the vertical and horizontal directions of the base to collect the vibration signals of the rotor, which are transmitted by the HG-8902 data acquisition box. Six types of faults were considered in the experiment: rotor unbalance I (RU1), rotor unbalance III (RU3), rotor unbalance V (RU5), rotor unbalance VII (RU7), fan broken blades (PPB) and base Loose (PL). In the specific diagnosis, the normal state (N) and these 6 faults will be distinguished together. The first four types of faults are simulated by installing different numbers of screws on the rotor. For example, installing 5 screws means that...
PUM
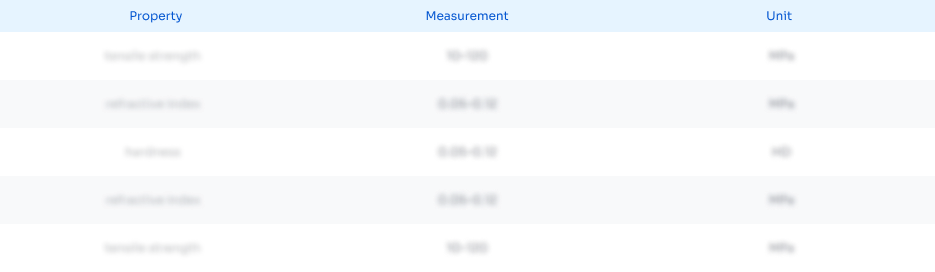
Abstract
Description
Claims
Application Information
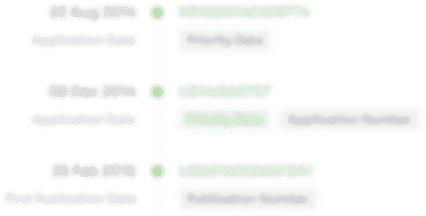
- R&D Engineer
- R&D Manager
- IP Professional
- Industry Leading Data Capabilities
- Powerful AI technology
- Patent DNA Extraction
Browse by: Latest US Patents, China's latest patents, Technical Efficacy Thesaurus, Application Domain, Technology Topic.
© 2024 PatSnap. All rights reserved.Legal|Privacy policy|Modern Slavery Act Transparency Statement|Sitemap