Cross-domain semantic segmentation method based on graph convolution and contrast learning
A semantic segmentation and convolution technology, applied in the field of pattern recognition and computer vision, can solve the problems of difficult migration of category proportion distribution, poor cross-domain effect, imbalanced distribution of different categories, etc., to achieve excellent evaluation results and improve class imbalance. Effect
- Summary
- Abstract
- Description
- Claims
- Application Information
AI Technical Summary
Problems solved by technology
Method used
Image
Examples
Embodiment 1
[0058] Embodiment 1: as Figure 1-Figure 3 As shown, the cross-domain semantic segmentation method based on graph convolution and contrastive learning, the specific steps of the method are as follows:
[0059] S1: Select a network training data set, the network training data set includes a source domain data set and a target domain data set, wherein the source domain data set contains labels, and the target domain data set does not contain labels; the target domain data set includes training data sets and test set;
[0060] S2: Construct the basic network model for semantic segmentation. First, use the source domain dataset to train the basic network model for semantic segmentation. Use the basic network model for semantic segmentation trained by the source domain dataset as the basic network, and combine the source domain dataset and the target domain dataset together. sent to the basic network for training;
[0061] S3: Use the feature map output by the middle layer of the...
PUM
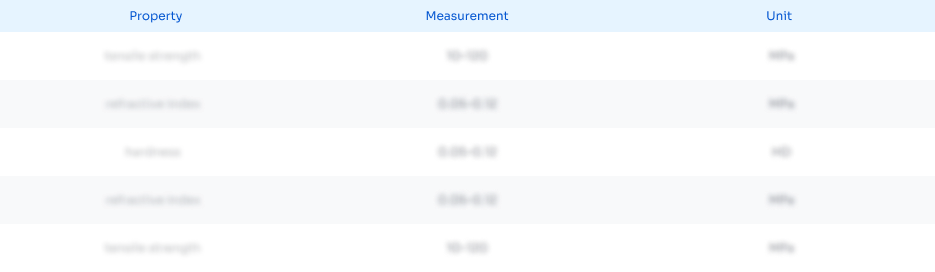
Abstract
Description
Claims
Application Information
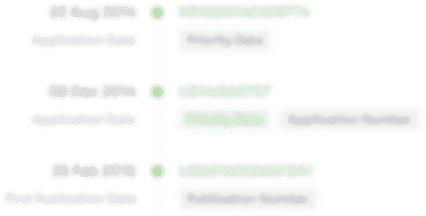
- R&D Engineer
- R&D Manager
- IP Professional
- Industry Leading Data Capabilities
- Powerful AI technology
- Patent DNA Extraction
Browse by: Latest US Patents, China's latest patents, Technical Efficacy Thesaurus, Application Domain, Technology Topic.
© 2024 PatSnap. All rights reserved.Legal|Privacy policy|Modern Slavery Act Transparency Statement|Sitemap