Systems and methods for processing MRI data
A data and preprocessing technology, applied in image data processing, application, image enhancement, etc., can solve the problem of long waiting time for MRI data acquisition and analysis
- Summary
- Abstract
- Description
- Claims
- Application Information
AI Technical Summary
Problems solved by technology
Method used
Image
Examples
example 1
[0094] Example 1: Parameter selection
[0095] In response to the limitations of conventional systems and methods for processing and / or preprocessing MRI data, the present disclosure provides an automated search method for selecting optimal fMRI preprocessing pipeline parameters. Embodiments of the disclosed systems and methods have been validated on two independent datasets.
[0096] For example, from two publicly available MRI datasets CNP LA5c1 (N = 251) and EMBARC2 (N = 330), MRI data were preprocessed using 72 different parameter sets. This is due to the disclosed technique's ability to perform parallel fMRI preprocessing at scale and through an AFNI-based cloud-enabled pipeline. These 72 parameter sets were created by varying four different parameters that would normally require manual optimization - two from the structure-function alignment step and two from the cranial stripping step.
[0097] For each of the 72 pipeline outputs per subject, a whole-brain functional ...
example 2
[0099] Example 2: Parallel processing for QC for parameter selection
[0100] In some embodiments of the present disclosure, preprocessing the received MRI data may include parallel processing. Preprocessing of structural and functional MRI scans is a computationally intensive operation, typically requiring several hours per subject. This leads to excessive latency between MRI data acquisition and analysis, especially in large datasets with hundreds of subjects, and especially when calculations are performed using traditional computer infrastructure such as high-performance workstation units.
[0101] The present disclosure provides for cloud computing and / or massively parallel MRI preprocessing pipelines. Parallel preprocessing may include any suitable parallel processing technique. In some embodiments, the method provides preprocessing averaging over 150 scans per day. For example, in certain embodiments, a preprocessing pipeline can be constructed using FreeSurfer and th...
example 3
[0105] Example 3: Machine Learning with Automated QC
[0106] Over the past twenty-five (25) years, advances in the collection and analysis of functional magnetic resonance imaging (fMRI) data have enabled new insights into the brain basis of human health and disease. Individual behavioral changes can now be visualized at the neural level as patterns of connectivity between brain regions. Functional brain imaging thus enhances our understanding of clinical psychiatric disorders by revealing links between regional and network abnormalities and psychiatric symptoms.
[0107] Recent initial successes in the field have prompted the collection of larger datasets that require the use of fMRI to generate brain-based biomarkers to support the development of precision medicines. Despite methodological advances and enhancements in computing power, assessing fMRI scan quality remains a critical step in the analytical framework. Prior to analysis, expert reviewers visually inspected ind...
PUM
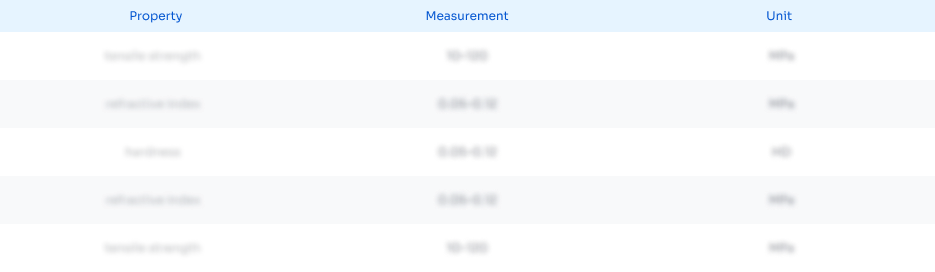
Abstract
Description
Claims
Application Information
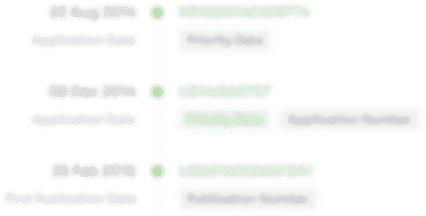
- R&D
- Intellectual Property
- Life Sciences
- Materials
- Tech Scout
- Unparalleled Data Quality
- Higher Quality Content
- 60% Fewer Hallucinations
Browse by: Latest US Patents, China's latest patents, Technical Efficacy Thesaurus, Application Domain, Technology Topic, Popular Technical Reports.
© 2025 PatSnap. All rights reserved.Legal|Privacy policy|Modern Slavery Act Transparency Statement|Sitemap|About US| Contact US: help@patsnap.com