Abnormal time series data value processing method based on adaptive threshold stationary wavelet transform
An adaptive threshold and time series data technology, applied in the field of signal processing, can solve the problems of reduced efficiency of abnormal data detection, reduced detection performance, false detection, etc., to achieve high abnormal value detection probability, low abnormal value false detection probability, high abnormal value detection probability The effect of detection probability
- Summary
- Abstract
- Description
- Claims
- Application Information
AI Technical Summary
Problems solved by technology
Method used
Image
Examples
Embodiment Construction
[0032] The embodiments and effects of the present invention will be further described in detail below in conjunction with the accompanying drawings.
[0033] refer to figure 1 , the implementation steps of the present invention are as follows:
[0034] Step 1. Obtain the time series data f(n) to be processed, and set the wavelet transform basis function and the number of wavelet transform layers m.
[0035] Obtain the time series data f(n) containing outliers to be processed from the time series data that needs to deal with outliers, and calculate its data length, assuming it is N;
[0036] In wavelet transform, since wavelet transform base functions have their own characteristics when processing data, when using wavelet transform to detect outliers, it is necessary to select wavelet functions similar to abrupt waveforms as wavelet transform base functions, which are currently commonly used in outlier detection The wavelet transform base functions include dbN series wavelet ...
PUM
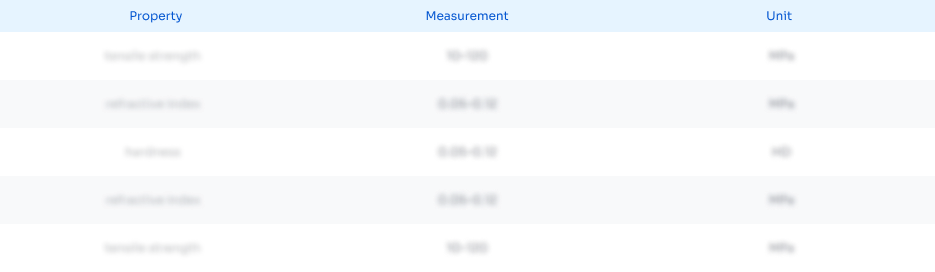
Abstract
Description
Claims
Application Information
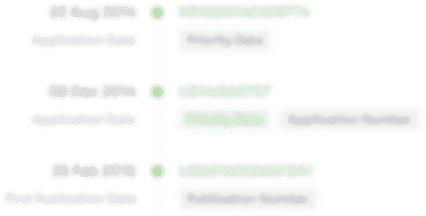
- R&D Engineer
- R&D Manager
- IP Professional
- Industry Leading Data Capabilities
- Powerful AI technology
- Patent DNA Extraction
Browse by: Latest US Patents, China's latest patents, Technical Efficacy Thesaurus, Application Domain, Technology Topic, Popular Technical Reports.
© 2024 PatSnap. All rights reserved.Legal|Privacy policy|Modern Slavery Act Transparency Statement|Sitemap|About US| Contact US: help@patsnap.com