Vehicle fuel economy evaluation method based on data analysis
A fuel economy and data analysis technology, applied in the field of vehicle-machine systems, can solve the problems of parameters and other parameters and weight influences, lack of long-term dynamic improvement capabilities, and poor pertinence, so as to improve calculation and operation efficiency and enhance learning The effect of the mechanism
- Summary
- Abstract
- Description
- Claims
- Application Information
AI Technical Summary
Problems solved by technology
Method used
Image
Examples
Embodiment 1
[0085] Step S1: Data processing, analyzing the original message data sent by the vehicle terminal to obtain the driving time, vehicle VIN code, load, engine speed, engine fuel flow, instantaneous fuel consumption, vehicle speed, percentage of net engine output torque, and accelerator pedal opening , clutch status and brake status data;
[0086] Step S2: If it is judged that it is a training mode, then step S3, step S4 and step S5 are executed in sequence;
[0087] Step S3: Construct a training data set and store it in a configuration file;
[0088] Step S4: Fill the training data into the fuel economy model based on the KNN model;
[0089] Step S5: Deploy the model.
[0090] In the step S3, the training data set is constructed and stored in the configuration file. In order to reduce the amount of data used as the training data set and optimize the efficiency of the model, the travel data is cut between small areas and the average value of the interval is calculated. The spec...
Embodiment 2
[0097] Step S1: Data processing, analyzing the original message data sent by the vehicle terminal to obtain the driving time, vehicle VIN code, load, engine speed, engine fuel flow, instantaneous fuel consumption, vehicle speed, percentage of net engine output torque, and accelerator pedal opening , clutch status and brake status data;
[0098] Step S2: If it is judged that it is not the training mode, execute step S6;
[0099] Step S6: Calculation of the actual fuel index of the trip;
[0100] Step S7: Fuel model, output fuel consumption prediction results and driving conditions;
[0101] Step S8: judge whether it is an economical trip according to the fuel consumption prediction result output in step S7, if yes, execute step S9; if not, execute step S10 and end;
[0102] Step S9: Carry out incremental learning;
[0103] Step S10: the non-incremental learning ends.
[0104] In the step S6, the calculation of the actual fuel index of the itinerary is based on the processed...
Embodiment 3
[0120] The fuel model outputs the driving conditions, the specific steps are as follows:
[0121] Extraction of driving conditions: Based on the original message data sent by the vehicle terminal analyzed in step S1, according to the acceleration, accelerator opening, brake clutch status, minimum duration of the working condition, maximum interval of the same working condition, gear position, engine Speed, to identify the working condition of the travel data.
[0122] The driving conditions identified by this method include:
[0123] Acceleration, deceleration, rapid acceleration, rapid deceleration, constant speed, start, stop, small throttle, medium throttle, large throttle, full throttle, idle speed, coasting, shifting, stepping on the brake, stepping on the clutch, near the external characteristic curve, low speed full throttle, High gear at low vehicle speed, low gear at high vehicle speed, skip shifting, rapid acceleration at start.
[0124] Step S11: Make a driving be...
PUM
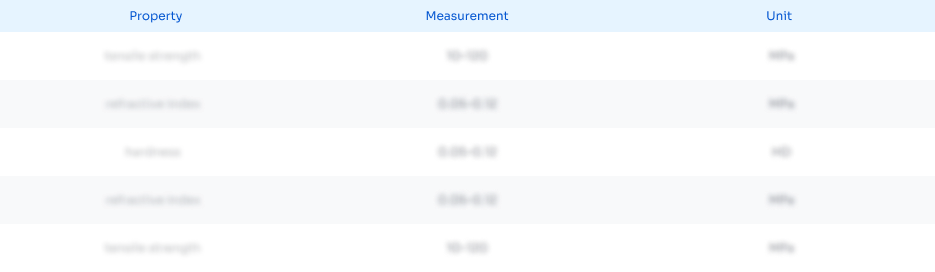
Abstract
Description
Claims
Application Information
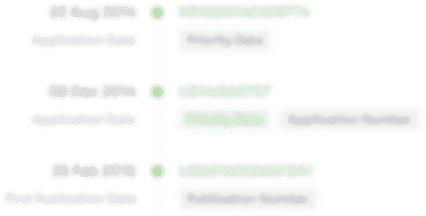
- R&D Engineer
- R&D Manager
- IP Professional
- Industry Leading Data Capabilities
- Powerful AI technology
- Patent DNA Extraction
Browse by: Latest US Patents, China's latest patents, Technical Efficacy Thesaurus, Application Domain, Technology Topic, Popular Technical Reports.
© 2024 PatSnap. All rights reserved.Legal|Privacy policy|Modern Slavery Act Transparency Statement|Sitemap|About US| Contact US: help@patsnap.com