Multi-agent game AI design method based on attention mechanism and reinforcement learning
A reinforcement learning, multi-agent technology, applied in the field of multi-agent deep reinforcement learning, can solve the problems of increasing complexity of the final strategy fusion process and insufficient strategy fusion, and achieve the effect of improving learning efficiency
- Summary
- Abstract
- Description
- Claims
- Application Information
AI Technical Summary
Problems solved by technology
Method used
Image
Examples
Embodiment Construction
[0017] The technical solution of the present invention will be described in detail below in conjunction with the accompanying drawings and specific embodiments.
[0018] The multi-agent game AI design method based on attention mechanism and reinforcement learning of the present invention includes division of local observation information and fusion of local strategies. like figure 1 Shown is the overall flowchart of the multi-agent game AI design method based on the attention mechanism and reinforcement learning of the present invention. The specific process is as follows:
[0019] Step 1. Divide and group the original observation information of the agent based on entity-based local information, specifically including the following processing:
[0020] Two entity observation features that are similar to entity observation features in the agent's field of view are divided into the same area in the entire field of view to form a group. The entity observation feature is the em...
PUM
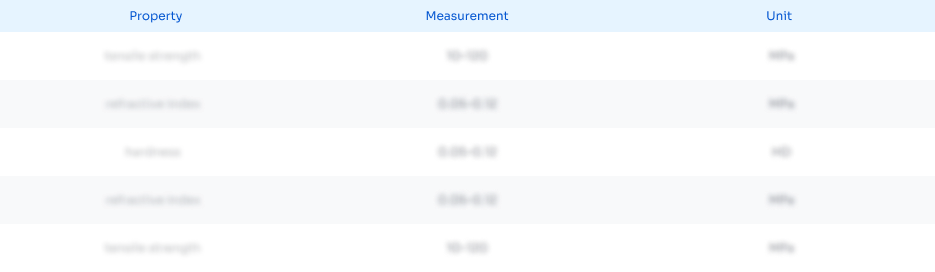
Abstract
Description
Claims
Application Information
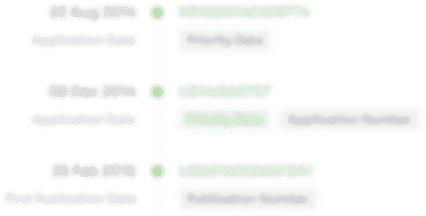
- R&D Engineer
- R&D Manager
- IP Professional
- Industry Leading Data Capabilities
- Powerful AI technology
- Patent DNA Extraction
Browse by: Latest US Patents, China's latest patents, Technical Efficacy Thesaurus, Application Domain, Technology Topic.
© 2024 PatSnap. All rights reserved.Legal|Privacy policy|Modern Slavery Act Transparency Statement|Sitemap