Task planning method based on symbol option and action model self-learning
A task planning and self-learning technology, applied in character and pattern recognition, computational models, machine learning, etc., can solve problems such as difficulty in adapting to high-dimensional environments, avoid repeated training, improve exploration efficiency, and improve data efficiency.
- Summary
- Abstract
- Description
- Claims
- Application Information
AI Technical Summary
Problems solved by technology
Method used
Image
Examples
Embodiment Construction
[0043] The present invention will be further described in detail below in conjunction with the accompanying drawings and specific embodiments. For the step numbers in the following embodiments, it is only set for the convenience of illustration and description, and the order between the steps is not limited in any way, and the execution order of each step in the embodiments can be adapted according to the understanding of those skilled in the art sexual adjustment.
[0044] refer to figure 1 with figure 2 , the present invention provides a task planning method based on symbolic options and action model self-learning, the method comprising the following steps:
[0045] Based on the symbolic state mapping module, high-dimensional image data is mapped to symbolic states according to prior knowledge and digital image processing;
[0046] Specifically, the symbolic state mapping module realizes the mapping from unstructured data to symbolic states composed of propositions based...
PUM
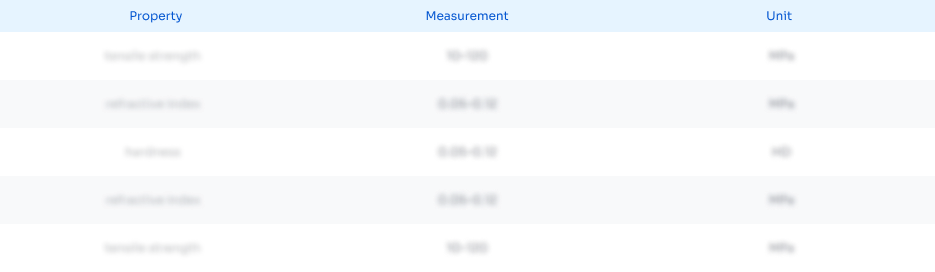
Abstract
Description
Claims
Application Information
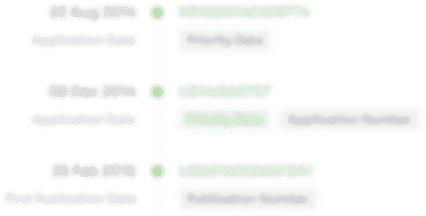
- R&D
- Intellectual Property
- Life Sciences
- Materials
- Tech Scout
- Unparalleled Data Quality
- Higher Quality Content
- 60% Fewer Hallucinations
Browse by: Latest US Patents, China's latest patents, Technical Efficacy Thesaurus, Application Domain, Technology Topic, Popular Technical Reports.
© 2025 PatSnap. All rights reserved.Legal|Privacy policy|Modern Slavery Act Transparency Statement|Sitemap|About US| Contact US: help@patsnap.com