Privacy protection method for knowledge migration in distributed machine learning
A machine learning and privacy protection technology, applied in the field of machine learning, can solve problems such as large time overhead, leakage, unprotected aggregated data, etc., and achieve the effects of ensuring security, efficient size comparison, time overhead and communication overhead
- Summary
- Abstract
- Description
- Claims
- Application Information
AI Technical Summary
Problems solved by technology
Method used
Image
Examples
Embodiment Construction
[0017] The present invention proposes a privacy protection method for knowledge transfer in distributed machine learning. Each client first trains its own teacher model, and the service requester provides unlabeled public data to the teacher model for prediction. The cloud server is safe The voting results of the teacher model are aggregated, and the corresponding labels are given through the security comparison algorithm. The service requester uses the samples with the given labels to train to obtain its own student model. During the whole training process, the cloud server did not directly access any user data.
[0018] combine figure 1 , a privacy protection method for knowledge transfer in distributed machine learning, the specific steps are:
[0019] Step 1. The client trains the teacher model, and uses the local data set to train its own teacher model;
[0020] Step 2. Aggregate labels. The service requester provides public data without labels to the client. Each teach...
PUM
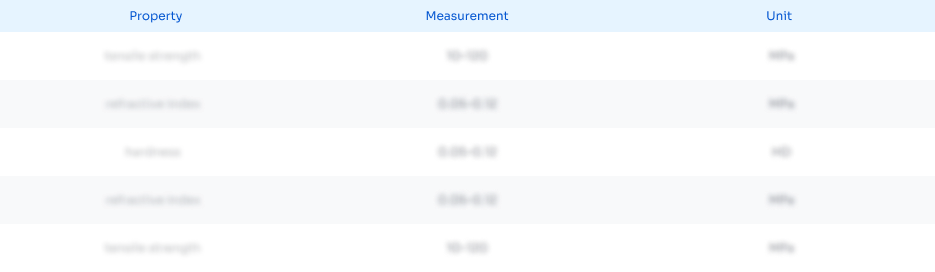
Abstract
Description
Claims
Application Information
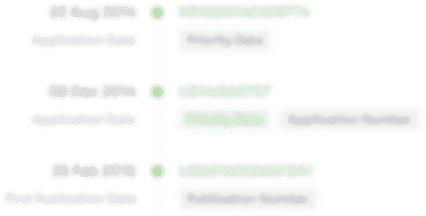
- R&D Engineer
- R&D Manager
- IP Professional
- Industry Leading Data Capabilities
- Powerful AI technology
- Patent DNA Extraction
Browse by: Latest US Patents, China's latest patents, Technical Efficacy Thesaurus, Application Domain, Technology Topic.
© 2024 PatSnap. All rights reserved.Legal|Privacy policy|Modern Slavery Act Transparency Statement|Sitemap