Laparoscopic surgery stage identification method and system based on dual-granularity time convolution
A recognition method and double-grained technology, applied in neural learning methods, character and pattern recognition, image data processing, etc., can solve problems such as difficult to accurately distinguish transition frames between stages, and achieve good recognition effect, improved recognition effect, and good generalization effect of ability
- Summary
- Abstract
- Description
- Claims
- Application Information
AI Technical Summary
Problems solved by technology
Method used
Image
Examples
Embodiment 1
[0085] Such as Figure 1 to Figure 4 As shown, a double-granularity temporal convolution-based laparoscopic surgery stage recognition method disclosed in this embodiment, the specific circumstances are as follows:
[0086] 1) First, the laparoscopic minimally invasive surgery process is recorded through a micro-camera installed at the tip of the surgical instrument, and each complete surgical process is saved as a video. Then use ffpmeg to slice each video, and save a picture every 5 frames, arranged in order of frame number. Then remove abnormal pictures, including pictures with problems such as full-image blur, large-scale phantoms, extreme lighting, and incomplete shooting, to make a data set for the laparoscopic surgery stage, and split it into training at a ratio of 40:8:32. set, validation set, and test set. Finally, OpenCV is used to perform image enhancement operations such as center flip, random cropping, and shuffle order on the laparoscopic surgery images.
[008...
Embodiment 2
[0119] see Figure 5 As shown, this embodiment discloses a laparoscopic surgery stage recognition system based on double-granularity time convolution, including the following functional modules:
[0120] The data acquisition module is used to collect laparoscopic surgery videos, down-sample each video, and retain several images at each stage of each video to make a laparoscopic surgery data set, according to "address / video serial number / frame serial number" The format named permutation forms a video sequence;
[0121] The data processing module is used to input the video sequence in the laparoscopic surgery data set into the first part of the double-granularity temporal convolution network, that is, the dual-granularity temporal convolution module, to model the long-distance temporal context information and generate the initial prediction result, And use the cross-entropy loss function to calculate the gap between the initial prediction result and the actual data; input the i...
PUM
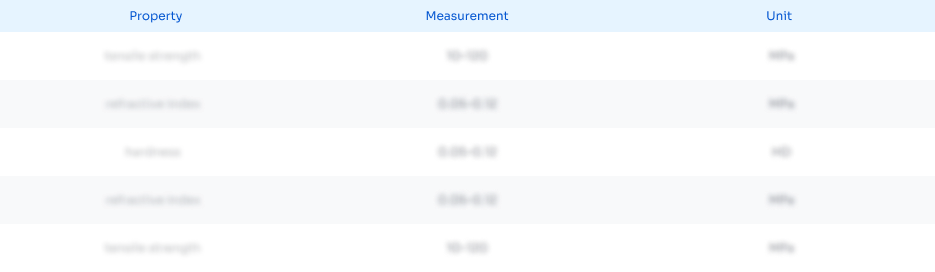
Abstract
Description
Claims
Application Information
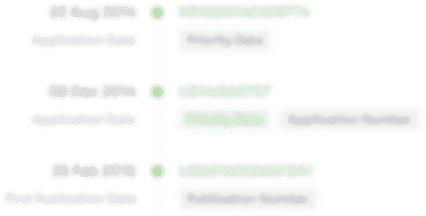
- R&D Engineer
- R&D Manager
- IP Professional
- Industry Leading Data Capabilities
- Powerful AI technology
- Patent DNA Extraction
Browse by: Latest US Patents, China's latest patents, Technical Efficacy Thesaurus, Application Domain, Technology Topic, Popular Technical Reports.
© 2024 PatSnap. All rights reserved.Legal|Privacy policy|Modern Slavery Act Transparency Statement|Sitemap|About US| Contact US: help@patsnap.com