Design and training of binary neurons and binary neural networks using error correction codes
A neural network, error correction technology, applied in the field of deep neural network, can solve the problem of no proposed training method and so on
- Summary
- Abstract
- Description
- Claims
- Application Information
AI Technical Summary
Problems solved by technology
Method used
Image
Examples
Embodiment Construction
[0061] The present invention proposes a purely binary neural network by introducing an architecture based on binary domain operations, and transforms the training problem into a communication channel decoding problem.
[0062] Artificial neurons have many inputs and weights, and return one output. It is usually thought of as a function parameterized by weights that takes the input of a neuron as a variable and returns an output. In the context of BNNs, of particular interest is the development of binary neurons whose inputs, weights, and outputs are all binary numbers. Interestingly, such a structure of binary neurons conforms to Boolean functions.
[0063] Let m be the number of variables, and is a binary m-tuple. Boolean function is to take F 2 Any function of values in f(x)=f(x 1 ,x 2 ,...,x m ). It can be defined by all 2 m A truth table specification of the value of f at input combinations. Table 1 gives an example of a truth table for a binary sum function (...
PUM
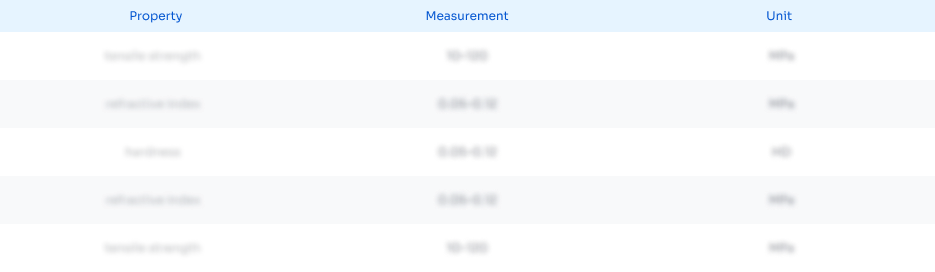
Abstract
Description
Claims
Application Information
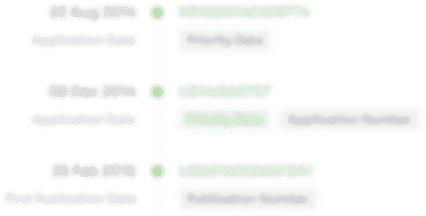
- R&D
- Intellectual Property
- Life Sciences
- Materials
- Tech Scout
- Unparalleled Data Quality
- Higher Quality Content
- 60% Fewer Hallucinations
Browse by: Latest US Patents, China's latest patents, Technical Efficacy Thesaurus, Application Domain, Technology Topic, Popular Technical Reports.
© 2025 PatSnap. All rights reserved.Legal|Privacy policy|Modern Slavery Act Transparency Statement|Sitemap|About US| Contact US: help@patsnap.com