Chinese clinical phenotype fine-grained named entity recognition method and system
A named entity recognition and fine-grained technology, applied in the field of clinical medical record information processing, can solve problems such as misleading analysis results
- Summary
- Abstract
- Description
- Claims
- Application Information
AI Technical Summary
Problems solved by technology
Method used
Image
Examples
Embodiment 1
[0037] The present embodiment 1 provides a Chinese clinical phenotype fine-grained named entity recognition system, which includes:
[0038] The extraction module is used for character-level embedded feature extraction of clinical text through the natural language pre-training model BERT;
[0039] The encoding module is used to integrate and encode the character-level embedded features and the sequence features of clinical texts by using the bidirectional long and short word memory model BiLSTM to obtain labels;
[0040] The decoding module is used to decode and predict the label by using the conditional random field CRF, and obtain the named entity recognition result.
[0041]In this embodiment 1, the above-mentioned system is used to realize a Chinese clinical phenotype fine-grained named entity recognition method, and the method includes:
[0042] Character-level embedding feature extraction of clinical text through natural language pre-training model BERT;
[0043] Using...
Embodiment 2
[0055] In this embodiment 2, a fine-grained phenotype named entity recognition method Phenonizer based on Chinese clinical medical records is provided, as follows figure 1 shown. In the framework of this method, the natural language pre-training model BERT is used to extract the character-level embedded features of clinical texts, and then the bidirectional long and short word memory model BiLSTM is used to integrate and encode the character-level features and text sequence features. The airport CRF performs the decoding prediction of the label.
[0056] In this embodiment 2, the Phenonizer technical framework includes three layers of text information processing modules, and the specific process is described as follows:
[0057] 1) Character-level embedding representation based on BERT layer
[0058] The input data of the natural language pre-training model BERT method is the patient's clinical medical record text sequence.
[0059] BERT is a pretrained language representat...
Embodiment 3
[0091] Embodiment 3 of the present invention provides an electronic device, including a memory and a processor, the processor and the memory communicate with each other, the memory stores program instructions that can be executed by the processor, and the processor invokes the The described program instruction executes the Chinese clinical phenotype fine-grained named entity recognition method, and the method includes the following process steps:
[0092] Character-level embedding feature extraction of clinical text through natural language pre-training model BERT;
[0093] Using the bidirectional long and short word memory model BiLSTM to integrate the character-level embedded features and the sequence features of clinical texts and encode the features to obtain labels;
[0094] The conditional random field (CRF) is used to decode and predict the label, and the named entity recognition result is obtained.
PUM
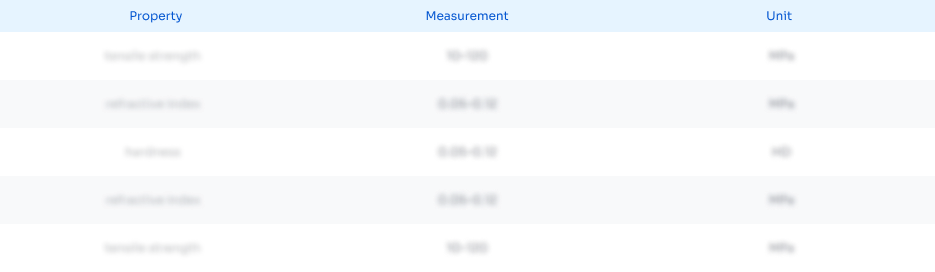
Abstract
Description
Claims
Application Information
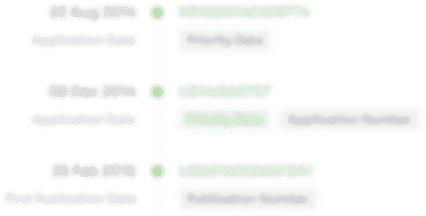
- R&D Engineer
- R&D Manager
- IP Professional
- Industry Leading Data Capabilities
- Powerful AI technology
- Patent DNA Extraction
Browse by: Latest US Patents, China's latest patents, Technical Efficacy Thesaurus, Application Domain, Technology Topic.
© 2024 PatSnap. All rights reserved.Legal|Privacy policy|Modern Slavery Act Transparency Statement|Sitemap