Distributed optical fiber vibration and sound wave sensing signal identification method based on SCNN
A distributed optical fiber and signal recognition technology, applied in character and pattern recognition, neural learning methods, measurement of ultrasonic/sonic/infrasonic waves, etc., can solve difficult online recognition of DVS/DAS sensing signals, poor actual system recognition ability, Long training and testing time, etc., achieve strong transfer learning ability and generalization ability, facilitate online real-time processing, and reduce the effect of calculation parameters
- Summary
- Abstract
- Description
- Claims
- Application Information
AI Technical Summary
Problems solved by technology
Method used
Image
Examples
Embodiment 1
[0036] Taking the long-distance pipeline safety monitoring application as an example, the processing flow of the distributed optical fiber vibration and acoustic wave sensing signal recognition (including feature extraction and classification) method based on pulsed convolutional neural network (SCNN) is as follows. figure 1 shown, including the following steps:
[0037] Step 1: Data preparation. The distributed optical fiber acoustic wave and vibration sensing system hardware based on phase sensitive optical time domain reflectometer is used to collect the acoustic wave or vibration signal (ie distributed optical fiber sensing signal) along the pipeline under the multi-scene complex background environment of the actual application site to construct the signal database , which includes a typical consistent signal data set A collected in the same scene, a typical inconsistent signal data set B and atypical inconsistent signal data set C collected in a small number of different ...
Embodiment 2
[0041] Further, the present invention is based on the distributed optical fiber vibration / acoustic wave sensing system (DVS / DAS) of linear phase demodulation (that is, the distributed optical fiber acoustic wave and vibration sensing system hardware based on the phase-sensitive optical time domain reflectometer) to realize long distance. Pipeline safety monitoring, the hardware structure and working principle of the application system used to collect sound waves or vibration signals along the pipeline under the multi-scene and complex background environment of the actual application site are as follows: figure 2 shown. The application system includes three parts: detection optical cable, optical signal demodulation equipment and signal processing host; the detection optical cable usually adopts ordinary single-mode communication optical fiber, which is buried along underground pipelines, power transmission cables and urban roads, and can also be directly used along pipelines. ...
Embodiment 3
[0046] The one-dimensional time series of each spatial point in the accumulated space-time signal matrix XX is divided into event signals along the time axis by column in turn, and the time series of its central space point is taken to construct a typical event signal dataset. In the present invention, the long-distance pipeline safety monitoring is taken as an example to construct a typical event signal data set related to pipeline safety. The specific operation process is as follows: For the signal time series of each spatial point, intercept the event signal with time length L in turn, such as image 3 As shown in the rectangular box in the middle, the time series of the central space point is obtained as the event signal sample, and the intercepted signals are recorded as etc., of which, means round down, X 1 Indicates the first segment of the signal intercepted by the central space point on the time axis, and is labeled with the event type according to the actual even...
PUM
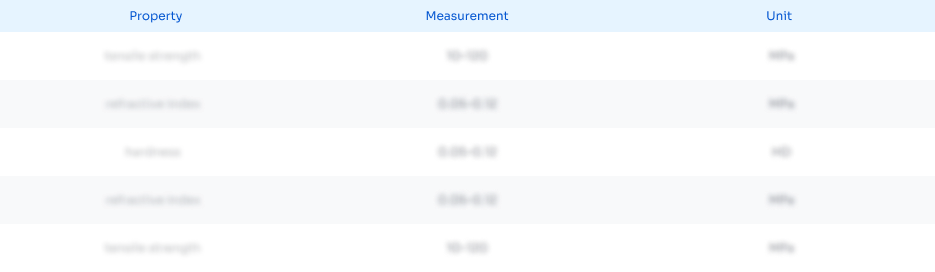
Abstract
Description
Claims
Application Information
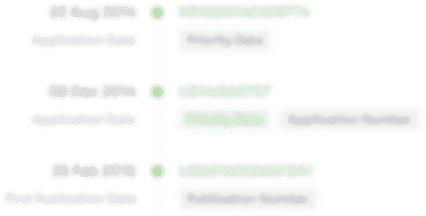
- R&D Engineer
- R&D Manager
- IP Professional
- Industry Leading Data Capabilities
- Powerful AI technology
- Patent DNA Extraction
Browse by: Latest US Patents, China's latest patents, Technical Efficacy Thesaurus, Application Domain, Technology Topic.
© 2024 PatSnap. All rights reserved.Legal|Privacy policy|Modern Slavery Act Transparency Statement|Sitemap