Road scene semantic segmentation method based on multi-model fusion
A semantic segmentation and multi-model technology, applied in the field of computer vision, can solve problems such as poor connectivity of segmentation results and insufficient accuracy of road category segmentation, so as to improve recognition accuracy and robustness, increase computing speed, improve accuracy and edge The effect of precision
- Summary
- Abstract
- Description
- Claims
- Application Information
AI Technical Summary
Benefits of technology
Problems solved by technology
Method used
Image
Examples
Embodiment Construction
[0077] In order to facilitate the understanding of those skilled in the art, the present invention will be further described below with reference to the embodiments and the accompanying drawings, and the contents mentioned in the embodiments are not intended to limit the present invention.
[0078] refer to figure 1 As shown, a method for semantic segmentation of road scenes based on multi-model fusion of the present invention, the steps are as follows:
[0079] 1) Build a multi-class model and a two-class model; specifically include:
[0080] 11) Building a multi-classification model based on an improved high-resolution network (HRNet); introducing visual attention, the multi-classification model outputs pixel-level label images, and predicts the category of pixels;
[0081] 12) Build a two-class model based on the codec structure of DeepLabV3+; the two-class model outputs the prediction result of the road category.
[0082] Wherein, the step 11) specifically includes:
[...
PUM
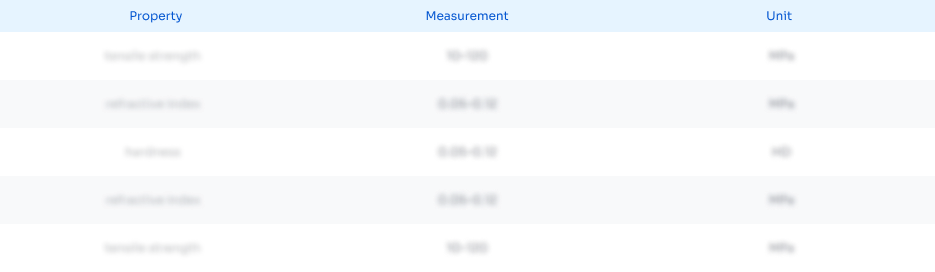
Abstract
Description
Claims
Application Information
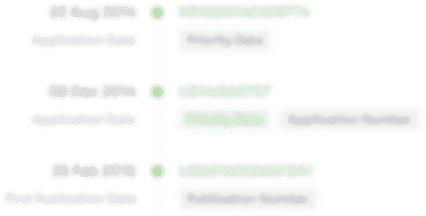
- Generate Ideas
- Intellectual Property
- Life Sciences
- Materials
- Tech Scout
- Unparalleled Data Quality
- Higher Quality Content
- 60% Fewer Hallucinations
Browse by: Latest US Patents, China's latest patents, Technical Efficacy Thesaurus, Application Domain, Technology Topic, Popular Technical Reports.
© 2025 PatSnap. All rights reserved.Legal|Privacy policy|Modern Slavery Act Transparency Statement|Sitemap|About US| Contact US: help@patsnap.com