Unsupervised anomaly detection method, system and device and storage medium
An anomaly detection and unsupervised technology, applied in the field of equipment and storage media, systems, and unsupervised anomaly detection methods, can solve the problems of poor detection effect, difficult migration, and difficult transformation into detection, and achieve high accuracy, good model robustness, etc. awesome effect
- Summary
- Abstract
- Description
- Claims
- Application Information
AI Technical Summary
Problems solved by technology
Method used
Image
Examples
Embodiment 1
[0034] The embodiment of the present invention provides an unsupervised anomaly detection method, which mainly includes: constructing a single-teacher multi-student unsupervised anomaly detection network assisted by semantic segmentation, including: a single teacher network, multiple student networks and a semantic segmentation network; All the student networks separately extract the multi-scale feature maps of the images to be tested, and fuse the multi-scale feature maps extracted by all the student networks according to the scale to obtain the fusion feature map of each scale; the multi-scale feature maps extracted by the teacher network According to the scale, it is compared with the fusion feature map of the corresponding scale to obtain the abnormal heat map of the corresponding scale, and the fusion result of the multi-scale abnormal heat map is obtained by combining the abnormal heat maps of all scales; the multi-scale abnormal heat map is analyzed by the semantic segmen...
Embodiment 2
[0101] The present invention also provides an unsupervised anomaly detection system, which is mainly implemented based on the methods provided in the foregoing embodiments, such as Figure 4 As shown, the system mainly includes:
[0102] Anomaly detection network construction unit, used to construct a single-teacher multi-student unsupervised anomaly detection network assisted by semantic segmentation, including: a single teacher network, multiple student networks and semantic segmentation networks;
[0103] The anomaly detection unit uses the constructed semantic segmentation-assisted single-teacher multi-student unsupervised anomaly detection network to perform anomaly detection. The steps include: separately extracting the multi-scale feature maps of the images to be tested through the teacher network and all the student networks, and all the student networks. The extracted multi-scale feature maps are fused according to the scale to obtain the fusion feature map of each sc...
Embodiment 3
[0106] The present invention also provides a processing device, such as Figure 5 As shown, it mainly includes: one or more processors; a memory for storing one or more programs; wherein, when the one or more programs are executed by the one or more processors, the One or more processors implement the methods provided by the foregoing embodiments.
[0107] Further, the processing device further includes at least one input device and at least one output device; in the processing device, the processor, the memory, the input device, and the output device are connected through a bus.
[0108] In this embodiment of the present invention, the specific types of the memory, the input device, and the output device are not limited; for example:
[0109] The input device can be a touch screen, an image capture device, a physical button or a mouse, etc.;
[0110] The output device can be a display terminal;
[0111] The memory may be random access memory (Random Access Memory, RAM), or...
PUM
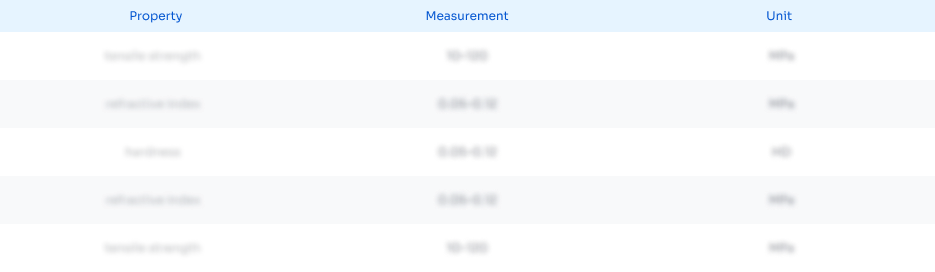
Abstract
Description
Claims
Application Information
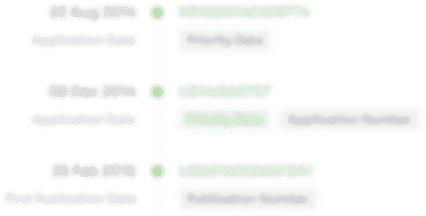
- R&D Engineer
- R&D Manager
- IP Professional
- Industry Leading Data Capabilities
- Powerful AI technology
- Patent DNA Extraction
Browse by: Latest US Patents, China's latest patents, Technical Efficacy Thesaurus, Application Domain, Technology Topic, Popular Technical Reports.
© 2024 PatSnap. All rights reserved.Legal|Privacy policy|Modern Slavery Act Transparency Statement|Sitemap|About US| Contact US: help@patsnap.com