Building vector extraction model based on deep learning and extraction method thereof
A technology of deep learning and extraction methods, applied in neural learning methods, biological neural network models, character and pattern recognition, etc., can solve the problems of shallow feature extraction and restoration, insufficient mining of image data features, and impact extraction As a result, follow-up applications and other issues have achieved the effect of enhancing information extraction capabilities, facilitating editing and application, and ensuring effectiveness.
- Summary
- Abstract
- Description
- Claims
- Application Information
AI Technical Summary
Problems solved by technology
Method used
Image
Examples
Embodiment Construction
[0054] The present invention will be described in further detail below with reference to the accompanying drawings and specific embodiments.
[0055] like figure 1 As shown, this embodiment discloses a new building vector extraction model based on dense convolutional neural network, including an encoder module 2, an optimization module 3, a decoder module 4 and a post-processing module 5. Based on this model, the The methods of building vector extraction include:
[0056] S1. The preprocessing module 1 performs preprocessing on the high-resolution remote sensing image including remote sensing image cropping, data enhancement and normalization. The encoder module 2 receives the preprocessed remote sensing image, and performs multiple stages of features on the remote sensing image. Extraction to obtain extracted features of multiple scales, and output features of one scale at each stage;
[0057] S2. The optimization module 3 respectively performs feature optimization on the e...
PUM
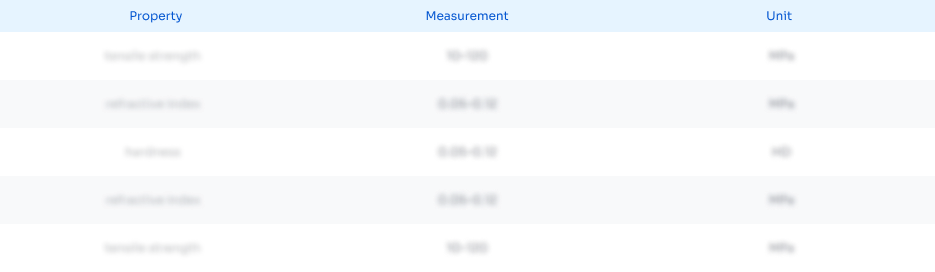
Abstract
Description
Claims
Application Information
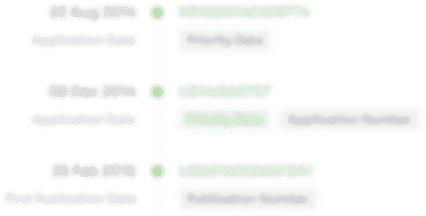
- R&D
- Intellectual Property
- Life Sciences
- Materials
- Tech Scout
- Unparalleled Data Quality
- Higher Quality Content
- 60% Fewer Hallucinations
Browse by: Latest US Patents, China's latest patents, Technical Efficacy Thesaurus, Application Domain, Technology Topic, Popular Technical Reports.
© 2025 PatSnap. All rights reserved.Legal|Privacy policy|Modern Slavery Act Transparency Statement|Sitemap|About US| Contact US: help@patsnap.com