Particle filtering resampling method suitable for non-linear probabilistic system posture
A non-linear random and system state technology, applied in the field of filtering, can solve the problems of poor state filtering accuracy, achieve the effect of improving filtering accuracy and overcoming poor filtering accuracy
- Summary
- Abstract
- Description
- Claims
- Application Information
AI Technical Summary
Problems solved by technology
Method used
Image
Examples
specific Embodiment approach 1
[0034] Embodiment 1: The particle filter resampling method applicable to the state of nonlinear stochastic system in this embodiment will be described in detail below.
[0035] The state-space model of the nonlinear stochastic system is as follows:
[0036] x k =f(x k-1 , u k-1 )(system model) (1)
[0037] the y k =h(x k , v k )(observation equation) (2)
[0038] Among them, f(.) and h(.) are known nonlinear functions, u k and v k are the system noise and observation noise with known probability density function, x k is the system state at time k, y k is k time x k observation value;
[0039] Order y k Represents the observed value of the real state of the system at time k, {x k (i): i=1,...,N} represents the state sample set at time k, {x * k (i): i=1,...,N} represents from {x k (i): The set of state samples obtained by resampling in i=1,...,N}, {x' k (i): i=1,...,N} represents a set of state samples, where
[0040] x' k (i)=f(x k-1 (i), 0), i=1, 2, .., N...
specific Embodiment approach 2
[0068] Embodiment 2: The difference between this embodiment and Embodiment 1 is that in step 2, the Pearson correlation coefficient function between two vectors or the angle function between two vectors is used as a function to measure the similarity of two vectors. When using the Pearson correlation coefficient function, the value range of s(i) is -1≤s(i)≤1, and when using the angle function, the value range of s(i) is 0≤s(i)≤π .
[0069] When this method is applied to the following representative nonlinear systems with bimodal characteristics of the observation likelihood function, the system state filtering accuracy is better than that of SIR, APF, RPF and GPF algorithms.
[0070] x k = x k - 1 2 + 25 x k - ...
PUM
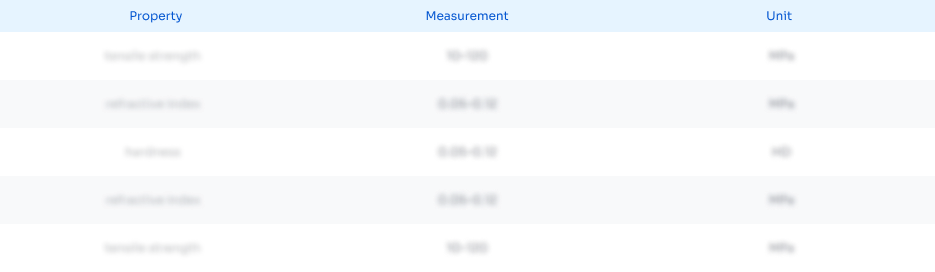
Abstract
Description
Claims
Application Information
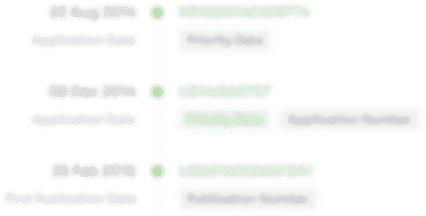
- R&D Engineer
- R&D Manager
- IP Professional
- Industry Leading Data Capabilities
- Powerful AI technology
- Patent DNA Extraction
Browse by: Latest US Patents, China's latest patents, Technical Efficacy Thesaurus, Application Domain, Technology Topic.
© 2024 PatSnap. All rights reserved.Legal|Privacy policy|Modern Slavery Act Transparency Statement|Sitemap