Adaptive modeling method for support vector regression based on KKT condition and nearest neighbor method
A technology of support vector regression and nearest neighbor method, which is applied in character and pattern recognition, computing models, computer components, etc., can solve the problems that static models cannot reflect the truth, and achieve the effect of reducing training time and storage space
- Summary
- Abstract
- Description
- Claims
- Application Information
AI Technical Summary
Problems solved by technology
Method used
Image
Examples
Embodiment Construction
[0024] A support vector regression machine adaptive modeling method based on KKT (Karush-Kuhn-Tucker) condition and nearest neighbor method, comprising the steps:
[0025] (1) Obtain the prior steady-state sample set D={x through off-line steady-state test 1 , x 2 ,...,x L}, and use the sample set D as the initial training sample set for model construction and learning, and pre-set the maximum capacity M of the initial training sample set;
[0026] (2) Let the first sample x k It is a new sample outside the sample set D, standardize the new sample, and judge whether it meets the KKT condition. Only the sample that violates the KKT condition contains new information and may become a new support vector. If the KKT condition is met, Then go to step (5), otherwise go to step (3);
[0027] (3) Establish a rolling time window with a width of L, and find the relationship with the first sample x by the following (A) formula k The second sample with the highest similarity x p , a...
PUM
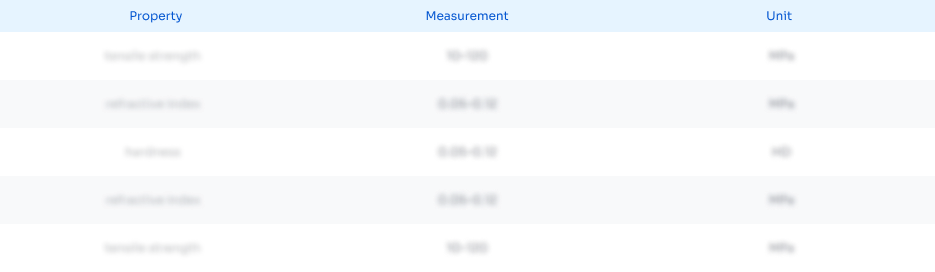
Abstract
Description
Claims
Application Information
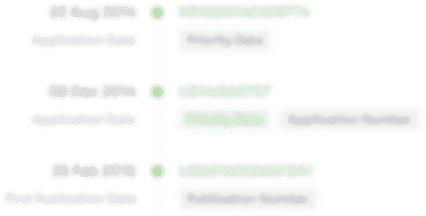
- R&D Engineer
- R&D Manager
- IP Professional
- Industry Leading Data Capabilities
- Powerful AI technology
- Patent DNA Extraction
Browse by: Latest US Patents, China's latest patents, Technical Efficacy Thesaurus, Application Domain, Technology Topic, Popular Technical Reports.
© 2024 PatSnap. All rights reserved.Legal|Privacy policy|Modern Slavery Act Transparency Statement|Sitemap|About US| Contact US: help@patsnap.com