Genetic evolution image rebuilding method based on Ridgelet redundant dictionary
A redundant dictionary and image reconstruction technology, which is applied in image enhancement, image data processing, genetic models, etc., can solve the problems of inaccurate reconstruction results, poor robustness, and no theoretical support, etc. Structural efficiency, weakening block effect and noise interference, and reducing time complexity
- Summary
- Abstract
- Description
- Claims
- Application Information
AI Technical Summary
Problems solved by technology
Method used
Image
Examples
Embodiment 1
[0077] The present invention is a genetic evolutionary image reconstruction method based on Ridgelet redundant dictionary. Signal reconstruction is a means to restore incomplete signals, the last link of compressed sensing (CS), and the core of compressed sensing theory. And key, image reconstruction is also a kind of signal reconstruction. In order to realize image reconstruction in the present invention, what needs to be found is a redundant base dictionary with good sparse representation performance and a solution for L 0 An effective method for the NP-hard problem of norm combination optimization, thus the present invention establishes a genetic evolution compressed sensing reconstruction method based on Ridgelet redundant dictionary. The first part of this method is to use the information of similar image blocks in the image, select the affine propagation AP algorithm that is insensitive to the cluster center and the number of categories to cluster and group all the image...
Embodiment 2
[0124] The genetic evolution image reconstruction method based on Ridgelet redundant dictionary is the same as embodiment 1, combined figure 1 with figure 2 , the specific implementation process of the present invention is described in detail as follows:
[0125] Step 1: Cluster the observation vectors
[0126] For the observation vectors of all image blocks sent by the image sender, the affine propagation clustering AP algorithm is used to group similar observation vectors together to obtain observation vectors of multiple categories.
[0127] In this embodiment, the 512×512 image is divided into 16×16 image blocks to obtain 1024 image blocks; all image blocks are saved as column vectors, and the column vectors corresponding to all image blocks are multiplied by the Gaussian observation matrix to obtain 1024 observation vector.
[0128] The specific steps of the affine propagation clustering AP algorithm are:
[0129] The first step is to set the moment when the number o...
Embodiment 3
[0198] The genetic evolution image reconstruction method based on Ridgelet redundant dictionary is the same as embodiment 1-2,
[0199] The present invention and the reconstruction effect comparison simulation experiment of existing OMP algorithm:
[0200] The purpose of the experiment is to solve the L 0 The present invention and the existing OMP algorithm compress the sensing reconstruction effect of the norm problem. The experimental test data are two standard test images Barbara and Lena with a size of 512×512, both of which adopt the idea of blocking for Gaussian observations, wherein the initial value of the image block sparsity of the present invention is set to 32, self-adaptive adjustment during evolution, evolution algebra is 5, while the image block sparsity in the OMP algorithm is fixed at 32. Table 2 lists the comparison of PSNR value of reconstructed images by two compressed sensing reconstruction methods. The Barbara diagram is based on the data comparison...
PUM
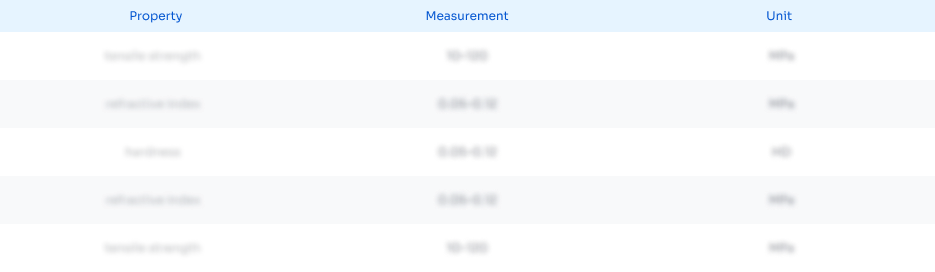
Abstract
Description
Claims
Application Information
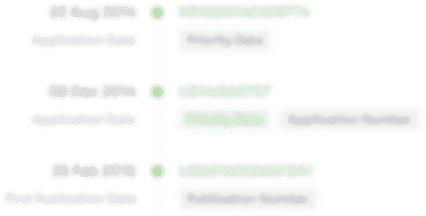
- R&D
- Intellectual Property
- Life Sciences
- Materials
- Tech Scout
- Unparalleled Data Quality
- Higher Quality Content
- 60% Fewer Hallucinations
Browse by: Latest US Patents, China's latest patents, Technical Efficacy Thesaurus, Application Domain, Technology Topic, Popular Technical Reports.
© 2025 PatSnap. All rights reserved.Legal|Privacy policy|Modern Slavery Act Transparency Statement|Sitemap|About US| Contact US: help@patsnap.com