Electronic nose data mining method based on supervised explicit manifold learning algorithm
A manifold learning and data mining technology, applied in electrical digital data processing, special data processing applications, computing, etc., can solve the error of electronic nose mode discrimination, the inability of electronic nose system to apply gas detection, and the inability to newly collect data for dimensionality reduction, etc. problem, to achieve the effect of high discrimination accuracy
- Summary
- Abstract
- Description
- Claims
- Application Information
AI Technical Summary
Problems solved by technology
Method used
Image
Examples
Embodiment Construction
[0043]The present invention will be further described below in combination with specific embodiments and accompanying drawings. The specific embodiments described here are only used to explain the present invention, not to limit the present invention.
[0044] In the embodiment, the electronic nose system is used for the diagnosis of wound infection, which mainly involves common clinical wound pathogen infection. The explicit manifold learning algorithm of the present invention adopts locality preserving projections (Locality Preserving Projections, LPP), and the supervised manifold learning algorithm adopts supervised locality preserving projections (Supervised Locality Preserving Projections, S-LPP).
[0045] In an embodiment of the present invention, the electronic nose data mining method based on LPP comprises the following steps:
[0046] Step 1. Collection of gas samples
[0047] The sensor array of the electronic nose system used in this example is composed of 15 gas ...
PUM
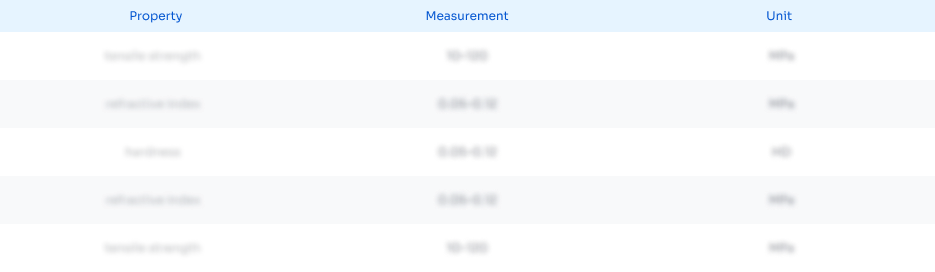
Abstract
Description
Claims
Application Information
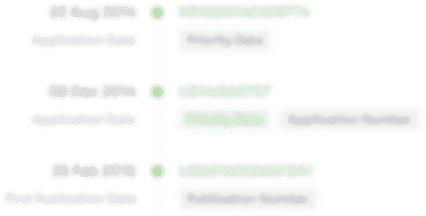
- Generate Ideas
- Intellectual Property
- Life Sciences
- Materials
- Tech Scout
- Unparalleled Data Quality
- Higher Quality Content
- 60% Fewer Hallucinations
Browse by: Latest US Patents, China's latest patents, Technical Efficacy Thesaurus, Application Domain, Technology Topic, Popular Technical Reports.
© 2025 PatSnap. All rights reserved.Legal|Privacy policy|Modern Slavery Act Transparency Statement|Sitemap|About US| Contact US: help@patsnap.com